Open Access
ARTICLE
Enhanced Steganalysis for Color Images Using Curvelet Features and Support Vector Machine
1 Faculty of Computer Science and Information Technology, Department of Computer Science, Superior University, Lahore, 54000, Pakistan
2 Machine Learning Code (MLC) Lab, Meharban House, House Number 209, Zafar Colony, Okara, 56300, Pakistan
3 Information Technology Services, University of Okara, Okara, 56310, Pakistan
4 Department of Computer Science & Engineering, University of Engineering & Technology Lahore, Narowal Campus, Narowal, 51601, Pakistan
5 Department of Computer Science and Software Engineering, Al Ain University, Al Ain, 11671, Abu Dhabi
6 Department of Computer Science, King Khalid University, Abha, 61421, Saudi Arabia
* Corresponding Author: Mubbashar Saddique. Email:
(This article belongs to the Special Issue: The Next Generation of Artificial Intelligence and the Intelligent Internet of Things)
Computers, Materials & Continua 2024, 78(1), 1311-1328. https://doi.org/10.32604/cmc.2023.040512
Received 21 March 2023; Accepted 06 December 2023; Issue published 30 January 2024
Abstract
Algorithms for steganography are methods of hiding data transfers in media files. Several machine learning architectures have been presented recently to improve stego image identification performance by using spatial information, and these methods have made it feasible to handle a wide range of problems associated with image analysis. Images with little information or low payload are used by information embedding methods, but the goal of all contemporary research is to employ high-payload images for classification. To address the need for both low- and high-payload images, this work provides a machine-learning approach to steganography image classification that uses Curvelet transformation to efficiently extract characteristics from both type of images. Support Vector Machine (SVM), a commonplace classification technique, has been employed to determine whether the image is a stego or cover. The Wavelet Obtained Weights (WOW), Spatial Universal Wavelet Relative Distortion (S-UNIWARD), Highly Undetectable Steganography (HUGO), and Minimizing the Power of Optimal Detector (MiPOD) steganography techniques are used in a variety of experimental scenarios to evaluate the performance of the proposed method. Using WOW at several payloads, the proposed approach proves its classification accuracy of 98.60%. It exhibits its superiority over SOTA methods.Keywords
Cite This Article
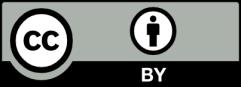
This work is licensed under a Creative Commons Attribution 4.0 International License , which permits unrestricted use, distribution, and reproduction in any medium, provided the original work is properly cited.