Open Access
ARTICLE
Asymmetric Loss Based on Image Properties for Deep Learning-Based Image Restoration
Henan Key Laboratory of Imaging and Intelligent Processing, PLA Strategic Support Force Information Engineering University, Zhengzhou, 450000, China
* Corresponding Author: Bin Yan. Email:
(This article belongs to the Special Issue: Advances and Applications in Signal, Image and Video Processing)
Computers, Materials & Continua 2023, 77(3), 3367-3386. https://doi.org/10.32604/cmc.2023.045878
Received 10 September 2023; Accepted 30 October 2023; Issue published 26 December 2023
Abstract
Deep learning techniques have significantly improved image restoration tasks in recent years. As a crucial component of deep learning, the loss function plays a key role in network optimization and performance enhancement. However, the currently prevalent loss functions assign equal weight to each pixel point during loss calculation, which hampers the ability to reflect the roles of different pixel points and fails to exploit the image’s characteristics fully. To address this issue, this study proposes an asymmetric loss function based on the image and data characteristics of the image recovery task. This novel loss function can adjust the weight of the reconstruction loss based on the grey value of different pixel points, thereby effectively optimizing the network training by differentially utilizing the grey information from the original image. Specifically, we calculate a weight factor for each pixel point based on its grey value and combine it with the reconstruction loss to create a new loss function. This ensures that pixel points with smaller grey values receive greater attention, improving network recovery. In order to verify the effectiveness of the proposed asymmetric loss function, we conducted experimental tests in the image super-resolution task. The experimental results show that the model with the introduction of asymmetric loss weights improves all the indexes of the processing results without increasing the training time. In the typical super-resolution network SRCNN, by introducing asymmetric weights, it is possible to improve the peak signal-to-noise ratio (PSNR) by up to about 0.5%, the structural similarity index (SSIM) by up to about 0.3%, and reduce the root-mean-square error (RMSE) by up to about 1.7% with essentially no increase in training time. In addition, we also further tested the performance of the proposed method in the denoising task to verify the potential applicability of the method in the image restoration task.Keywords
Cite This Article
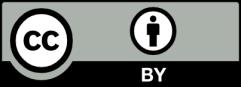