Open Access
ARTICLE
CFSA-Net: Efficient Large-Scale Point Cloud Semantic Segmentation Based on Cross-Fusion Self-Attention
1 School of Electrical and Engineering, Hubei University of Technology, Wuhan, 430068, China
2 Hubei Key Laboratory for High-Efficiency Utilization of Solar Energy and Operation Control of Energy Storage System, Hubei University of Technology, Wuhan, 430068, China
3 School of Mechanical and Electrical Engineering, Wuhan Donghu University, Wuhan, 430212, China
* Corresponding Author: Jie Zhang. Email:
Computers, Materials & Continua 2023, 77(3), 2677-2697. https://doi.org/10.32604/cmc.2023.045818
Received 08 September 2023; Accepted 10 November 2023; Issue published 26 December 2023
Abstract
Traditional models for semantic segmentation in point clouds primarily focus on smaller scales. However, in real-world applications, point clouds often exhibit larger scales, leading to heavy computational and memory requirements. The key to handling large-scale point clouds lies in leveraging random sampling, which offers higher computational efficiency and lower memory consumption compared to other sampling methods. Nevertheless, the use of random sampling can potentially result in the loss of crucial points during the encoding stage. To address these issues, this paper proposes cross-fusion self-attention network (CFSA-Net), a lightweight and efficient network architecture specifically designed for directly processing large-scale point clouds. At the core of this network is the incorporation of random sampling alongside a local feature extraction module based on cross-fusion self-attention (CFSA). This module effectively integrates long-range contextual dependencies between points by employing hierarchical position encoding (HPC). Furthermore, it enhances the interaction between each point's coordinates and feature information through cross-fusion self-attention pooling, enabling the acquisition of more comprehensive geometric information. Finally, a residual optimization (RO) structure is introduced to extend the receptive field of individual points by stacking hierarchical position encoding and cross-fusion self-attention pooling, thereby reducing the impact of information loss caused by random sampling. Experimental results on the Stanford Large-Scale 3D Indoor Spaces (S3DIS), Semantic3D, and SemanticKITTI datasets demonstrate the superiority of this algorithm over advanced approaches such as RandLA-Net and KPConv. These findings underscore the excellent performance of CFSA-Net in large-scale 3D semantic segmentation.Keywords
Cite This Article
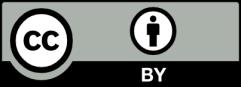
This work is licensed under a Creative Commons Attribution 4.0 International License , which permits unrestricted use, distribution, and reproduction in any medium, provided the original work is properly cited.