Open Access
ARTICLE
Bearing Fault Diagnosis with DDCNN Based on Intelligent Feature Fusion Strategy in Strong Noise
1 College of Electronic Information and Optical Engineering, Taiyuan University of Technology, Taiyuan, 030024, China
2 Key Lab of Advanced Transducers and Intelligent Control System of the Ministry of Education, Taiyuan, 030024, China
* Corresponding Author: Runfang Hao. Email:
(This article belongs to the Special Issue: Trends in Machine Learning and Internet of Things for Industrial Applications)
Computers, Materials & Continua 2023, 77(3), 3423-3442. https://doi.org/10.32604/cmc.2023.045718
Received 05 September 2023; Accepted 04 November 2023; Issue published 26 December 2023
Abstract
Intelligent fault diagnosis in modern mechanical equipment maintenance is increasingly adopting deep learning technology. However, conventional bearing fault diagnosis models often suffer from low accuracy and unstable performance in noisy environments due to their reliance on a single input data. Therefore, this paper proposes a dual-channel convolutional neural network (DDCNN) model that leverages dual data inputs. The DDCNN model introduces two key improvements. Firstly, one of the channels substitutes its convolution with a larger kernel, simplifying the structure while addressing the lack of global information and shallow features. Secondly, the feature layer combines data from different sensors based on their primary and secondary importance, extracting details through small kernel convolution for primary data and obtaining global information through large kernel convolution for secondary data. Extensive experiments conducted on two-bearing fault datasets demonstrate the superiority of the two-channel convolution model, exhibiting high accuracy and robustness even in strong noise environments. Notably, it achieved an impressive 98.84% accuracy at a Signal to Noise Ratio (SNR) of −4 dB, outperforming other advanced convolutional models.Keywords
Cite This Article
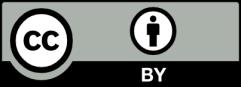