Open Access
ARTICLE
An Adaptive DDoS Detection and Classification Method in Blockchain Using an Integrated Multi-Models
1 School of Computer Science and Technology, Hainan University, Haikou, 570228, China
2 School of Cyberspace Security, Hainan University, Haikou, 570228, China
3 Hainan Blockchain Technology Engineering Research Center, Hainan University, Haikou, 570228, China
4 Hainan Hairui Zhong Chuang Technol Co., Ltd., Haikou, 570228, China
5 School of Information Science and Technology, Qiongtai Normal University, Haikou, 570228, China
* Corresponding Author: Jieren Cheng. Email:
Computers, Materials & Continua 2023, 77(3), 3265-3288. https://doi.org/10.32604/cmc.2023.045588
Received 31 August 2023; Accepted 03 November 2023; Issue published 26 December 2023
Abstract
With the rising adoption of blockchain technology due to its decentralized, secure, and transparent features, ensuring its resilience against network threats, especially Distributed Denial of Service (DDoS) attacks, is crucial. This research addresses the vulnerability of blockchain systems to DDoS assaults, which undermine their core decentralized characteristics, posing threats to their security and reliability. We have devised a novel adaptive integration technique for the detection and identification of varied DDoS attacks. To ensure the robustness and validity of our approach, a dataset amalgamating multiple DDoS attacks was derived from the CIC-DDoS2019 dataset. Using this, our methodology was applied to detect DDoS threats and further classify them into seven unique attack subcategories. To cope with the broad spectrum of DDoS attack variations, a holistic framework has been proposed that seamlessly integrates five machine learning models: Gate Recurrent Unit (GRU), Convolutional Neural Networks (CNN), Long-Short Term Memory (LSTM), Deep Neural Networks (DNN), and Support Vector Machine (SVM). The innovative aspect of our framework is the introduction of a dynamic weight adjustment mechanism, enhancing the system’s adaptability. Experimental results substantiate the superiority of our ensemble method in comparison to singular models across various evaluation metrics. The framework displayed remarkable accuracy, with rates reaching 99.71% for detection and 87.62% for classification tasks. By developing a comprehensive and adaptive methodology, this study paves the way for strengthening the defense mechanisms of blockchain systems against DDoS attacks. The ensemble approach, combined with the dynamic weight adjustment, offers promise in ensuring blockchain’s enduring security and trustworthiness.Keywords
Cite This Article
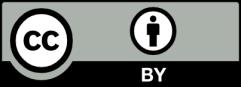
This work is licensed under a Creative Commons Attribution 4.0 International License , which permits unrestricted use, distribution, and reproduction in any medium, provided the original work is properly cited.