Open Access
ARTICLE
Enhancing Breast Cancer Diagnosis with Channel-Wise Attention Mechanisms in Deep Learning
School of Computer and Artificial Intelligence, Zhengzhou University, Zhengzhou, 450001, China
* Corresponding Author: Zhenfei Wang. Email:
(This article belongs to the Special Issue: Deep Learning in Medical Imaging-Disease Segmentation and Classification)
Computers, Materials & Continua 2023, 77(3), 2699-2714. https://doi.org/10.32604/cmc.2023.045310
Received 23 August 2023; Accepted 12 October 2023; Issue published 26 December 2023
Abstract
Breast cancer, particularly Invasive Ductal Carcinoma (IDC), is a primary global health concern predominantly affecting women. Early and precise diagnosis is crucial for effective treatment planning. Several AI-based techniques for IDC-level classification have been proposed in recent years. Processing speed, memory size, and accuracy can still be improved for better performance. Our study presents ECAM, an Enhanced Channel-Wise Attention Mechanism, using deep learning to analyze histopathological images of Breast Invasive Ductal Carcinoma (BIDC). The main objectives of our study are to enhance computational efficiency using a Separable CNN architecture, improve data representation through hierarchical feature aggregation, and increase accuracy and interpretability with channel-wise attention mechanisms. Utilizing publicly available datasets, DataBioX IDC and the BreakHis, we benchmarked the proposed ECAM model against existing state-of-the-art models: DenseNet121, VGG16, and AlexNet. In the IDC dataset, the model based on AlexNet achieved an accuracy rate of 86.81% and an F1 score of 86.94%. On the other hand, DenseNet121 outperformed with an accuracy of 95.60% and an F1 score of 95.75%. Meanwhile, the VGG16 model achieved an accuracy rate of 91.20% and an F1 score of 90%. Our proposed ECAM model outperformed the state-of-the-art, achieving an impressive F1 score of 96.65% and an accuracy rate of 96.70%. The BreakHis dataset, the AlexNet-based model, achieved an accuracy rate of 90.82% and an F1 score of 90.77%. DenseNet121 achieved a higher accuracy rate of 92.66% with an F1 score of 92.72%, while the VGG16 model achieved an accuracy of 92.60% and an F1 score of 91.31%. The proposed ECAM model again outperformed, achieving an F1 score of 96.37% and an accuracy rate of 96.33%. Our model is a significant advancement in breast cancer diagnosis, with high accuracy and potential as an automated grading, especially for IDC.Keywords
Cite This Article
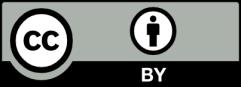
This work is licensed under a Creative Commons Attribution 4.0 International License , which permits unrestricted use, distribution, and reproduction in any medium, provided the original work is properly cited.