Open Access
ARTICLE
Feature-Based Augmentation in Sarcasm Detection Using Reverse Generative Adversarial Network
1 Computer Science Department, School of Computer Science, Bina Nusantara University, Jakarta, 11480, Indonesia
2 Cyber Security Program, Computer Science Department, School of Computer Science, Bina Nusantara University, Jakarta, 11480, Indonesia
* Corresponding Author: Derwin Suhartono. Email:
Computers, Materials & Continua 2023, 77(3), 3637-3657. https://doi.org/10.32604/cmc.2023.045301
Received 23 August 2023; Accepted 27 November 2023; Issue published 26 December 2023
Abstract
Sarcasm detection in text data is an increasingly vital area of research due to the prevalence of sarcastic content in online communication. This study addresses challenges associated with small datasets and class imbalances in sarcasm detection by employing comprehensive data pre-processing and Generative Adversial Network (GAN) based augmentation on diverse datasets, including iSarcasm, SemEval-18, and Ghosh. This research offers a novel pipeline for augmenting sarcasm data with Reverse Generative Adversarial Network (RGAN). The proposed RGAN method works by inverting labels between original and synthetic data during the training process. This inversion of labels provides feedback to the generator for generating high-quality data closely resembling the original distribution. Notably, the proposed RGAN model exhibits performance on par with standard GAN, showcasing its robust efficacy in augmenting text data. The exploration of various datasets highlights the nuanced impact of augmentation on model performance, with cautionary insights into maintaining a delicate balance between synthetic and original data. The methodological framework encompasses comprehensive data pre-processing and GAN-based augmentation, with a meticulous comparison against Natural Language Processing Augmentation (NLPAug) as an alternative augmentation technique. Overall, the F1-score of our proposed technique outperforms that of the synonym replacement augmentation technique using NLPAug. The increase in F1-score in experiments using RGAN ranged from 0.066% to 1.054%, and the use of standard GAN resulted in a 2.88% increase in F1-score. The proposed RGAN model outperformed the NLPAug method and demonstrated comparable performance to standard GAN, emphasizing its efficacy in text data augmentation.Keywords
Cite This Article
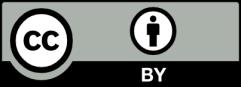
This work is licensed under a Creative Commons Attribution 4.0 International License , which permits unrestricted use, distribution, and reproduction in any medium, provided the original work is properly cited.