Open Access
ARTICLE
A Fusion of Residual Blocks and Stack Auto Encoder Features for Stomach Cancer Classification
1 Department of Computer Science, HITEC University, Taxila, 47080, Pakistan
2 Department of Computer Science and Mathematics, Lebanese American University, Beirut, 1100, Lebanon
3 College of Computer Science and Engineering, University of Ha’il, Ha’il, 81451, Saudi Arabia
4 Department of Information Systems, College of Computer and Information Sciences, Princess Nourah bint Abdulrahman University, P.O. Box 84428, Riyadh, Saudi Arabia
5 Department of Management Information Systems, College of Business Administration, Prince Sattam Bin Abdulaziz University, Al-Kharj, 16278, Saudi Arabia
6 Department of Computer Science, Hanyang University, Seoul, 04763, Korea
* Corresponding Author: Muhammad Attique Khan. Email:
(This article belongs to the Special Issue: Deep Learning in Medical Imaging-Disease Segmentation and Classification)
Computers, Materials & Continua 2023, 77(3), 3895-3920. https://doi.org/10.32604/cmc.2023.045244
Received 21 August 2023; Accepted 21 November 2023; Issue published 26 December 2023
Abstract
Diagnosing gastrointestinal cancer by classical means is a hazardous procedure. Years have witnessed several computerized solutions for stomach disease detection and classification. However, the existing techniques faced challenges, such as irrelevant feature extraction, high similarity among different disease symptoms, and the least-important features from a single source. This paper designed a new deep learning-based architecture based on the fusion of two models, Residual blocks and Auto Encoder. First, the Hyper-Kvasir dataset was employed to evaluate the proposed work. The research selected a pre-trained convolutional neural network (CNN) model and improved it with several residual blocks. This process aims to improve the learning capability of deep models and lessen the number of parameters. Besides, this article designed an Auto-Encoder-based network consisting of five convolutional layers in the encoder stage and five in the decoder phase. The research selected the global average pooling and convolutional layers for the feature extraction optimized by a hybrid Marine Predator optimization and Slime Mould optimization algorithm. These features of both models are fused using a novel fusion technique that is later classified using the Artificial Neural Network classifier. The experiment worked on the HyperKvasir dataset, which consists of 23 stomach-infected classes. At last, the proposed method obtained an improved accuracy of 93.90% on this dataset. Comparison is also conducted with some recent techniques and shows that the proposed method’s accuracy is improved.Keywords
Cite This Article
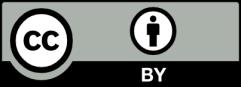
This work is licensed under a Creative Commons Attribution 4.0 International License , which permits unrestricted use, distribution, and reproduction in any medium, provided the original work is properly cited.