Open Access
ARTICLE
An Improved Multi-Objective Hybrid Genetic-Simulated Annealing Algorithm for AGV Scheduling under Composite Operation Mode
1 School of Transportation and Logistics Engineering, Wuhan University of Technology, Wuhan, 430063, China
2 Hubei Plog Technology Co., Ltd., Wuhan, 430000, China
* Corresponding Author: Xiaohua Cao. Email:
Computers, Materials & Continua 2023, 77(3), 3443-3466. https://doi.org/10.32604/cmc.2023.045120
Received 17 August 2023; Accepted 03 November 2023; Issue published 26 December 2023
Abstract
This paper presents an improved hybrid algorithm and a multi-objective model to tackle the scheduling problem of multiple Automated Guided Vehicles (AGVs) under the composite operation mode. The multi-objective model aims to minimize the maximum completion time, the total distance covered by AGVs, and the distance traveled while empty-loaded. The improved hybrid algorithm combines the improved genetic algorithm (GA) and the simulated annealing algorithm (SA) to strengthen the local search ability of the algorithm and improve the stability of the calculation results. Based on the characteristics of the composite operation mode, the authors introduce the combined coding and parallel decoding mode and calculate the fitness function with the grey entropy parallel analysis method to solve the multi-objective problem. The grey entropy parallel analysis method is a combination of the grey correlation analysis method and the entropy weighting method to solve multi-objective solving problems. A task advance evaluation strategy is proposed in the process of crossover and mutation operator to guide the direction of crossover and mutation. The computational experiments results show that the improved hybrid algorithm is better than the GA and the genetic algorithm with task advance evaluation strategy (AEGA) in terms of convergence speed and solution results, and the effectiveness of the multi-objective solution is proved. All three objectives are optimized and the proposed algorithm has an optimization of 7.6% respectively compared with the GA and 3.4% compared with the AEGA in terms of the objective of maximum completion time.Keywords
Cite This Article
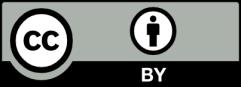
This work is licensed under a Creative Commons Attribution 4.0 International License , which permits unrestricted use, distribution, and reproduction in any medium, provided the original work is properly cited.