Open Access
ARTICLE
FIR-YOLACT: Fusion of ICIoU and Res2Net for YOLACT on Real-Time Vehicle Instance Segmentation
1 College of Big Data and Information Engineering, Guizhou University, Guiyang, 550025, China
2 The State Key Laboratory of Public Big Data, Guizhou University, Guiyang, 550025, China
* Corresponding Author: Ziyan Liu. Email:
Computers, Materials & Continua 2023, 77(3), 3551-3572. https://doi.org/10.32604/cmc.2023.044967
Received 13 August 2023; Accepted 03 November 2023; Issue published 26 December 2023
Abstract
Autonomous driving technology has made a lot of outstanding achievements with deep learning, and the vehicle detection and classification algorithm has become one of the critical technologies of autonomous driving systems. The vehicle instance segmentation can perform instance-level semantic parsing of vehicle information, which is more accurate and reliable than object detection. However, the existing instance segmentation algorithms still have the problems of poor mask prediction accuracy and low detection speed. Therefore, this paper proposes an advanced real-time instance segmentation model named FIR-YOLACT, which fuses the ICIoU (Improved Complete Intersection over Union) and Res2Net for the YOLACT algorithm. Specifically, the ICIoU function can effectively solve the degradation problem of the original CIoU loss function, and improve the training convergence speed and detection accuracy. The Res2Net module fused with the ECA (Efficient Channel Attention) Net is added to the model’s backbone network, which improves the multi-scale detection capability and mask prediction accuracy. Furthermore, the Cluster NMS (Non-Maximum Suppression) algorithm is introduced in the model’s bounding box regression to enhance the performance of detecting similarly occluded objects. The experimental results demonstrate the superiority of FIR-YOLACT to the based methods and the effectiveness of all components. The processing speed reaches 28 FPS, which meets the demands of real-time vehicle instance segmentation.Keywords
Cite This Article
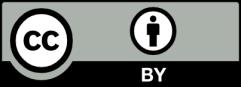
This work is licensed under a Creative Commons Attribution 4.0 International License , which permits unrestricted use, distribution, and reproduction in any medium, provided the original work is properly cited.