Open Access
ARTICLE
Improved Speech Emotion Recognition Focusing on High-Level Data Representations and Swift Feature Extraction Calculation
1 Department of Computer Engineering, Gachon University, Sujeong-Gu, Seongnam-Si, Gyeonggi-Do, 13120, Korea
2 Department of AI.Software, Gachon University, Seongnam-Si, 13120, Korea
3 Department of Artificial Intelligence, Tashkent State University of Economics, Tashkent, 100066, Uzbekistan
* Corresponding Author: Taeg Keun Whangbo. Email:
(This article belongs to the Special Issue: Advanced Artificial Intelligence and Machine Learning Frameworks for Signal and Image Processing Applications)
Computers, Materials & Continua 2023, 77(3), 2915-2933. https://doi.org/10.32604/cmc.2023.044466
Received 31 July 2023; Accepted 18 October 2023; Issue published 26 December 2023
Abstract
The performance of a speech emotion recognition (SER) system is heavily influenced by the efficacy of its feature extraction techniques. The study was designed to advance the field of SER by optimizing feature extraction techniques, specifically through the incorporation of high-resolution Mel-spectrograms and the expedited calculation of Mel Frequency Cepstral Coefficients (MFCC). This initiative aimed to refine the system’s accuracy by identifying and mitigating the shortcomings commonly found in current approaches. Ultimately, the primary objective was to elevate both the intricacy and effectiveness of our SER model, with a focus on augmenting its proficiency in the accurate identification of emotions in spoken language. The research employed a dual-strategy approach for feature extraction. Firstly, a rapid computation technique for MFCC was implemented and integrated with a Bi-LSTM layer to optimize the encoding of MFCC features. Secondly, a pretrained ResNet model was utilized in conjunction with feature Stats pooling and dense layers for the effective encoding of Mel-spectrogram attributes. These two sets of features underwent separate processing before being combined in a Convolutional Neural Network (CNN) outfitted with a dense layer, with the aim of enhancing their representational richness. The model was rigorously evaluated using two prominent databases: CMU-MOSEI and RAVDESS. Notable findings include an accuracy rate of 93.2% on the CMU-MOSEI database and 95.3% on the RAVDESS database. Such exceptional performance underscores the efficacy of this innovative approach, which not only meets but also exceeds the accuracy benchmarks established by traditional models in the field of speech emotion recognition.Keywords
Cite This Article
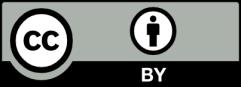
This work is licensed under a Creative Commons Attribution 4.0 International License , which permits unrestricted use, distribution, and reproduction in any medium, provided the original work is properly cited.