Open Access
ARTICLE
Detection of Safety Helmet-Wearing Based on the YOLO_CA Model
State Key Laboratory of Public Big Data, Guizhou University, Guiyang, 550025, China
* Corresponding Author: Songrong Qian. Email:
(This article belongs to the Special Issue: Deep Learning based Object Detection and Tracking in Videos)
Computers, Materials & Continua 2023, 77(3), 3349-3366. https://doi.org/10.32604/cmc.2023.043671
Received 09 July 2023; Accepted 10 October 2023; Issue published 26 December 2023
Abstract
Safety helmets can reduce head injuries from object impacts and lower the probability of safety accidents, as well as being of great significance to construction safety. However, for a variety of reasons, construction workers nowadays may not strictly enforce the rules of wearing safety helmets. In order to strengthen the safety of construction site, the traditional practice is to manage it through methods such as regular inspections by safety officers, but the cost is high and the effect is poor. With the popularization and application of construction site video monitoring, manual video monitoring has been realized for management, but the monitors need to be on duty at all times, and thus are prone to negligence. Therefore, this study establishes a lightweight model YOLO_CA based on YOLOv5 for the automatic detection of construction workers’ helmet wearing, which overcomes the shortcomings of the current manual monitoring methods that are inefficient and expensive. The coordinate attention (CA) addition to the YOLOv5 backbone strengthens detection accuracy in complex scenes by extracting critical information and suppressing non-critical information. Further parameter compression with deeply separable convolution (DWConv). In addition, to improve the feature representation speed, we swap out C3 with a Ghost module, which decreases the floating-point operations needed for feature channel fusion, and CIOU_Loss was substituted with EIOU_Loss to enhance the algorithm’s localization accuracy. Therefore, the original model needs to be improved so as to enhance the detection of safety helmets. The experimental results show that the YOLO_CA model achieves good results in all indicators compared with the mainstream model. Compared with the original model, the mAP value of the optimized model increased by 1.13%, GFLOPs cut down by 17.5%, and there is a 6.84% decrease in the total model parameters, furthermore, the weight size cuts down by 4.26%, FPS increased by 39.58%, and the detection effect and model size of this model can meet the requirements of lightweight embedding.Keywords
Cite This Article
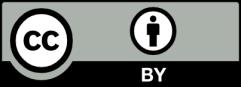
This work is licensed under a Creative Commons Attribution 4.0 International License , which permits unrestricted use, distribution, and reproduction in any medium, provided the original work is properly cited.