Open Access
ARTICLE
An Efficient Method for Identifying Lower Limb Behavior Intentions Based on Surface Electromyography
1 School of Artificial Intelligence, Anhui University of Science and Technology, Huainan, 232001, China
2 Institute of Environment-Friendly Materials and Occupational Health, Anhui University of Science and Technology, Wuhu, 241003, China
3 School of Electrical & Information Engineering, Anhui University of Science and Technology, Huainan, 232001, China
4 School of Mechanical Engineering, Anhui University of Science and Technology, Huainan, 232001, China
* Corresponding Author: Yiwen Wang. Email:
(This article belongs to the Special Issue: Advanced Artificial Intelligence and Machine Learning Frameworks for Signal and Image Processing Applications)
Computers, Materials & Continua 2023, 77(3), 2771-2790. https://doi.org/10.32604/cmc.2023.043383
Received 30 June 2023; Accepted 12 October 2023; Issue published 26 December 2023
Abstract
Surface electromyography (sEMG) is widely used for analyzing and controlling lower limb assisted exoskeleton robots. Behavior intention recognition based on sEMG is of great significance for achieving intelligent prosthetic and exoskeleton control. Achieving highly efficient recognition while improving performance has always been a significant challenge. To address this, we propose an sEMG-based method called Enhanced Residual Gate Network (ERGN) for lower-limb behavioral intention recognition. The proposed network combines an attention mechanism and a hard threshold function, while combining the advantages of residual structure, which maps sEMG of multiple acquisition channels to the lower limb motion states. Firstly, continuous wavelet transform (CWT) is used to extract signals features from the collected sEMG data. Then, a hard threshold function serves as the gate function to enhance signals quality, with an attention mechanism incorporated to improve the ERGN’s performance further. Experimental results demonstrate that the proposed ERGN achieves extremely high accuracy and efficiency, with an average recognition accuracy of 98.41% and an average recognition time of only 20 ms-outperforming the state-of-the-art research significantly. Our research provides support for the application of lower limb assisted exoskeleton robots.Keywords
Cite This Article
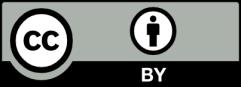
This work is licensed under a Creative Commons Attribution 4.0 International License , which permits unrestricted use, distribution, and reproduction in any medium, provided the original work is properly cited.