Open Access
ARTICLE
Multi-Equipment Detection Method for Distribution Lines Based on Improved YOLOx-s
1 School of Computer and Information Engineering, Jiangxi Normal University, Nanchang, 330022, China
2 School of Digital Industry, Jiangxi Normal University, Shangrao, 334005, China
3 Department of Science and Technology, State Grid Jiangxi Electric Power Research Institute, Nanchang, 330096, China
4 School of Information, North China University of Technology, Beijing, 100144, China
* Corresponding Author: Lei Hu. Email:
(This article belongs to the Special Issue: Machine Vision Detection and Intelligent Recognition)
Computers, Materials & Continua 2023, 77(3), 2735-2749. https://doi.org/10.32604/cmc.2023.042974
Received 18 June 2023; Accepted 12 October 2023; Issue published 26 December 2023
Abstract
The YOLOx-s network does not sufficiently meet the accuracy demand of equipment detection in the autonomous inspection of distribution lines by Unmanned Aerial Vehicle (UAV) due to the complex background of distribution lines, variable morphology of equipment, and large differences in equipment sizes. Therefore, aiming at the difficult detection of power equipment in UAV inspection images, we propose a multi-equipment detection method for inspection of distribution lines based on the YOLOx-s. Based on the YOLOx-s network, we make the following improvements: 1) The Receptive Field Block (RFB) module is added after the shallow feature layer of the backbone network to expand the receptive field of the network. 2) The Coordinate Attention (CA) module is added to obtain the spatial direction information of the targets and improve the accuracy of target localization. 3) After the first fusion of features in the Path Aggregation Network (PANet), the Adaptively Spatial Feature Fusion (ASFF) module is added to achieve efficient re-fusion of multi-scale deep and shallow feature maps by assigning adaptive weight parameters to features at different scales. 4) The loss function Binary Cross Entropy (BCE) Loss in YOLOx-s is replaced by Focal Loss to alleviate the difficulty of network convergence caused by the imbalance between positive and negative samples of small-sized targets. The experiments take a private dataset consisting of four types of power equipment: Transformers, Isolators, Drop Fuses, and Lightning Arrestors. On average, the mean Average Precision (mAP) of the proposed method can reach 93.64%, an increase of 3.27%. The experimental results show that the proposed method can better identify multiple types of power equipment of different scales at the same time, which helps to improve the intelligence of UAV autonomous inspection in distribution lines.Keywords
Cite This Article
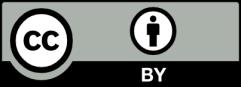
This work is licensed under a Creative Commons Attribution 4.0 International License , which permits unrestricted use, distribution, and reproduction in any medium, provided the original work is properly cited.