Open Access
ARTICLE
Joint On-Demand Pruning and Online Distillation in Automatic Speech Recognition Language Model Optimization
1 Clova Speech, Naver Corporation, Seongnam, 13561, Korea
2 Department of Computer Science and Engineering, Sogang University, Seoul, 04107, Korea
* Corresponding Author: Ji-Hwan Kim. Email:
Computers, Materials & Continua 2023, 77(3), 2833-2856. https://doi.org/10.32604/cmc.2023.042816
Received 13 June 2023; Accepted 13 October 2023; Issue published 26 December 2023
Abstract
Automatic speech recognition (ASR) systems have emerged as indispensable tools across a wide spectrum of applications, ranging from transcription services to voice-activated assistants. To enhance the performance of these systems, it is important to deploy efficient models capable of adapting to diverse deployment conditions. In recent years, on-demand pruning methods have obtained significant attention within the ASR domain due to their adaptability in various deployment scenarios. However, these methods often confront substantial trade-offs, particularly in terms of unstable accuracy when reducing the model size. To address challenges, this study introduces two crucial empirical findings. Firstly, it proposes the incorporation of an online distillation mechanism during on-demand pruning training, which holds the promise of maintaining more consistent accuracy levels. Secondly, it proposes the utilization of the Mogrifier long short-term memory (LSTM) language model (LM), an advanced iteration of the conventional LSTM LM, as an effective alternative for pruning targets within the ASR framework. Through rigorous experimentation on the ASR system, employing the Mogrifier LSTM LM and training it using the suggested joint on-demand pruning and online distillation method, this study provides compelling evidence. The results exhibit that the proposed methods significantly outperform a benchmark model trained solely with on-demand pruning methods. Impressively, the proposed strategic configuration successfully reduces the parameter count by approximately 39%, all the while minimizing trade-offs.Keywords
Cite This Article
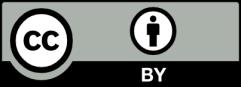
This work is licensed under a Creative Commons Attribution 4.0 International License , which permits unrestricted use, distribution, and reproduction in any medium, provided the original work is properly cited.