Open Access
ARTICLE
DL-Powered Anomaly Identification System for Enhanced IoT Data Security
Department Computer Science, Prince Sattam Bin Abdulaziz University, Wadi Ad-Dawaser, 11990, Saudi Arabia
* Corresponding Author: Manjur Kolhar. Email:
Computers, Materials & Continua 2023, 77(3), 2857-2879. https://doi.org/10.32604/cmc.2023.042726
Received 09 June 2023; Accepted 13 September 2023; Issue published 26 December 2023
Abstract
In many commercial and public sectors, the Internet of Things (IoT) is deeply embedded. Cyber security threats aimed at compromising the security, reliability, or accessibility of data are a serious concern for the IoT. Due to the collection of data from several IoT devices, the IoT presents unique challenges for detecting anomalous behavior. It is the responsibility of an Intrusion Detection System (IDS) to ensure the security of a network by reporting any suspicious activity. By identifying failed and successful attacks, IDS provides a more comprehensive security capability. A reliable and efficient anomaly detection system is essential for IoT-driven decision-making. Using deep learning-based anomaly detection, this study proposes an IoT anomaly detection system capable of identifying relevant characteristics in a controlled environment. These factors are used by the classifier to improve its ability to identify fraudulent IoT data. For efficient outlier detection, the author proposed a Convolutional Neural Network (CNN) with Long Short Term Memory (LSTM) based Attention Mechanism (ACNN-LSTM). As part of the ACNN-LSTM model, CNN units are deployed with an attention mechanism to avoid memory loss and gradient dispersion. Using the N-BaIoT and IoT-23 datasets, the model is verified. According to the N-BaIoT dataset, the overall accuracy is 99%, and precision, recall, and F1-score are also 0.99. In addition, the IoT-23 dataset shows a commendable accuracy of 99%. In terms of accuracy and recall, it scored 0.99, while the F1-score was 0.98. The LSTM model with attention achieved an accuracy of 95%, while the CNN model achieved an accuracy of 88%. According to the loss graph, attention-based models had lower loss values, indicating that they were more effective at detecting anomalies. In both the N-BaIoT and IoT-23 datasets, the receiver operating characteristic and area under the curve (ROC-AUC) graphs demonstrated exceptional accuracy of 99% to 100% for the Attention-based CNN and LSTM models. This indicates that these models are capable of making precise predictions.Keywords
Cite This Article
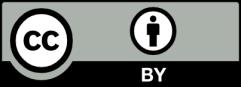
This work is licensed under a Creative Commons Attribution 4.0 International License , which permits unrestricted use, distribution, and reproduction in any medium, provided the original work is properly cited.