Open Access
ARTICLE
One Dimensional Conv-BiLSTM Network with Attention Mechanism for IoT Intrusion Detection
1 Department of Information Systems, Al-Farabi Kazakh National University, Almaty, Kazakhstan
2 Department of Information Technology and Security, Abylkas Saginov Karaganda Technical University, Karaganda, Kazakhstan
3 Department of Computer Science and Information Technology, K. Zhubanov Aktobe Regional University, Aktobe, Kazakhstan
4 Department of Information Systems, International Information Technology University, Almaty, Kazakhstan
5 Department of Computer Science, Korkyt Ata Kyzylorda State University, Kyzylorda, Kazakhstan
* Corresponding Author: Bauyrzhan Omarov. Email:
Computers, Materials & Continua 2023, 77(3), 3765-3781. https://doi.org/10.32604/cmc.2023.042469
Received 31 May 2023; Accepted 24 August 2023; Issue published 26 December 2023
Abstract
In the face of escalating intricacy and heterogeneity within Internet of Things (IoT) network landscapes, the imperative for adept intrusion detection techniques has never been more pressing. This paper delineates a pioneering deep learning-based intrusion detection model: the One Dimensional Convolutional Neural Networks (1D-CNN) and Bidirectional Long Short-Term Memory (BiLSTM) Network (Conv-BiLSTM) augmented with an Attention Mechanism. The primary objective of this research is to engineer a sophisticated model proficient in discerning the nuanced patterns and temporal dependencies quintessential to IoT network traffic data, thereby facilitating the precise categorization of a myriad of intrusion types. Methodology: The proposed model amalgamates the potent attributes of 1D convolutional neural networks, bidirectional long short-term memory layers, and attention mechanisms to bolster the efficacy and resilience of IoT intrusion detection systems. A rigorous assessment was executed employing an expansive dataset that mirrors the convolutions and multifariousness characteristic of genuine IoT network settings, encompassing various network traffic paradigms and intrusion archetypes. Findings: The empirical evidence underscores the paramountcy of the One Dimensional Conv-BiLSTM Network with Attention Mechanism, which exhibits a marked superiority over conventional machine learning modalities. Notably, the model registers an exemplary AUC-ROC metric of 0.995, underscoring its precision in typifying a spectrum of intrusions within IoT infrastructures. Conclusion: The presented One Dimensional Conv-BiLSTM Network armed with an Attention Mechanism stands out as a robust and trustworthy vanguard against IoT network breaches. Its prowess in discerning intricate traffic patterns and inherent temporal dependencies transcends that of traditional machine learning frameworks. The commendable diagnostic accuracy manifested in this study advocates for its tangible deployment. This investigation indubitably advances the cybersecurity domain, amplifying the fortification and robustness of IoT frameworks and heralding a new era of bolstered security across pivotal sectors such as residential, medical, and transit systems.Keywords
Cite This Article
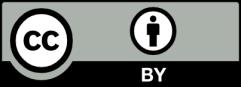
This work is licensed under a Creative Commons Attribution 4.0 International License , which permits unrestricted use, distribution, and reproduction in any medium, provided the original work is properly cited.