Open Access
ARTICLE
Smart MobiNet: A Deep Learning Approach for Accurate Skin Cancer Diagnosis
1 Department of Computer Science, Bacha Khan University, Charsadda, Pakistan
2 Department of Information Systems, College of Computer and Information Sciences, Princess Nourah bint Abdulrahman University, P.O. Box 84428, Riyadh, 11671, Saudi Arabia
3 Faculty of Computer Studies, Arab Open University, Muscat, Oman
4 School of Biochemistry and Biotechnology, University of the Punjab, Lahore, Pakistan
* Corresponding Author: Ghadah Aldehim. Email:
(This article belongs to the Special Issue: Advances, Challenges, and Opportunities of IoT-Based Big Data in Healthcare Industry 4.0)
Computers, Materials & Continua 2023, 77(3), 3533-3549. https://doi.org/10.32604/cmc.2023.042365
Received 27 May 2023; Accepted 03 November 2023; Issue published 26 December 2023
Abstract
The early detection of skin cancer, particularly melanoma, presents a substantial risk to human health. This study aims to examine the necessity of implementing efficient early detection systems through the utilization of deep learning techniques. Nevertheless, the existing methods exhibit certain constraints in terms of accessibility, diagnostic precision, data availability, and scalability. To address these obstacles, we put out a lightweight model known as Smart MobiNet, which is derived from MobileNet and incorporates additional distinctive attributes. The model utilizes a multi-scale feature extraction methodology by using various convolutional layers. The ISIC 2019 dataset, sourced from the International Skin Imaging Collaboration, is employed in this study. Traditional data augmentation approaches are implemented to address the issue of model overfitting. In this study, we conduct experiments to evaluate and compare the performance of three different models, namely CNN, MobileNet, and Smart MobiNet, in the task of skin cancer detection. The findings of our study indicate that the proposed model outperforms other architectures, achieving an accuracy of 0.89. Furthermore, the model exhibits balanced precision, sensitivity, and F1 scores, all measuring at 0.90. This model serves as a vital instrument that assists clinicians efficiently and precisely detecting skin cancer.Keywords
Cite This Article
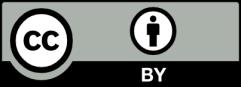
This work is licensed under a Creative Commons Attribution 4.0 International License , which permits unrestricted use, distribution, and reproduction in any medium, provided the original work is properly cited.