Open Access
ARTICLE
DNEF: A New Ensemble Framework Based on Deep Network Structure
1 College of Mathematics and Informatics, South China Agricultural University, Guangzhou, 510642, China
2 School of Statistics and Mathematics, Guangdong University of Finance and Economics, Guangzhou, 510120, China
* Corresponding Author: Ge Song. Email:
Computers, Materials & Continua 2023, 77(3), 4055-4072. https://doi.org/10.32604/cmc.2023.042277
Received 24 May 2023; Accepted 24 October 2023; Issue published 26 December 2023
Abstract
Deep neural networks have achieved tremendous success in various fields, and the structure of these networks is a key factor in their success. In this paper, we focus on the research of ensemble learning based on deep network structure and propose a new deep network ensemble framework (DNEF). Unlike other ensemble learning models, DNEF is an ensemble learning architecture of network structures, with serial iteration between the hidden layers, while base classifiers are trained in parallel within these hidden layers. Specifically, DNEF uses randomly sampled data as input and implements serial iteration based on the weighting strategy between hidden layers. In the hidden layers, each node represents a base classifier, and multiple nodes generate training data for the next hidden layer according to the transfer strategy. The DNEF operates based on two strategies: (1) The weighting strategy calculates the training instance weights of the nodes according to their weaknesses in the previous layer. (2) The transfer strategy adaptively selects each node’s instances with weights as transfer instances and transfer weights, which are combined with the training data of nodes as input for the next hidden layer. These two strategies improve the accuracy and generalization of DNEF. This research integrates the ensemble of all nodes as the final output of DNEF. The experimental results reveal that the DNEF framework surpasses the traditional ensemble models and functions with high accuracy and innovative deep ensemble methods.Keywords
Cite This Article
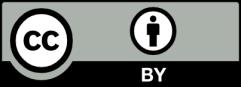
This work is licensed under a Creative Commons Attribution 4.0 International License , which permits unrestricted use, distribution, and reproduction in any medium, provided the original work is properly cited.