Open Access
REVIEW
Multi-Robot Privacy-Preserving Algorithms Based on Federated Learning: A Review
1 College of Automation, Guangxi University of Science and Technology, Liuzhou, 545000, China
2 Department of Artificial Intelligence and Manufacturing, Hechi University, Hechi, 547000, China
* Corresponding Author: Jiansheng Peng. Email:
Computers, Materials & Continua 2023, 77(3), 2971-2994. https://doi.org/10.32604/cmc.2023.041897
Received 05 May 2023; Accepted 18 October 2023; Issue published 26 December 2023
Abstract
The robotics industry has seen rapid development in recent years due to the Corona Virus Disease 2019. With the development of sensors and smart devices, factories and enterprises have accumulated a large amount of data in their daily production, which creates extremely favorable conditions for robots to perform machine learning. However, in recent years, people’s awareness of data privacy has been increasing, leading to the inability to circulate data between different enterprises, resulting in the emergence of data silos. The emergence of federated learning provides a feasible solution to this problem, and the combination of federated learning and multi-robot systems can break down data silos and improve the overall performance of robots. However, as scholars have studied more deeply, they found that federated learning has very limited privacy protection. Therefore, how to protect data privacy from infringement remains an important issue. In this paper, we first give a brief introduction to the current development of multi-robot and federated learning; second, we review three aspects of privacy protection methods commonly used, privacy protection methods for multi-robot, and Other Problems Faced by Multi-robot Systems, focusing on method comparisons and challenges; and finally draw conclusions and predict possible future research directions.Keywords
Cite This Article
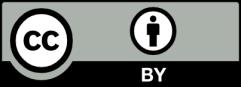
This work is licensed under a Creative Commons Attribution 4.0 International License , which permits unrestricted use, distribution, and reproduction in any medium, provided the original work is properly cited.