Open Access
ARTICLE
Multiclass Classification for Cyber Threats Detection on Twitter
1 College of Computer Science and Engineering, Department of Computer Science, AL-Ahgaff University, Mukalla, Yemen
2 College of Computing and Information Technology at Khulais, Department of Information Technology, University of Jeddah, Jeddah, Saudi Arabia
* Corresponding Author: Abdulwahab Ali Almazroi. Email:
Computers, Materials & Continua 2023, 77(3), 3853-3866. https://doi.org/10.32604/cmc.2023.040856
Received 01 April 2023; Accepted 25 July 2023; Issue published 26 December 2023
Abstract
The advances in technology increase the number of internet systems usage. As a result, cybersecurity issues have become more common. Cyber threats are one of the main problems in the area of cybersecurity. However, detecting cybersecurity threats is not a trivial task and thus is the center of focus for many researchers due to its importance. This study aims to analyze Twitter data to detect cyber threats using a multiclass classification approach. The data is passed through different tasks to prepare it for the analysis. Term Frequency and Inverse Document Frequency (TFIDF) features are extracted to vectorize the cleaned data and several machine learning algorithms are used to classify the Twitter posts into multiple classes of cyber threats. The results are evaluated using different metrics including precision, recall, F-score, and accuracy. This work contributes to the cyber security research area. The experiments revealed the promised results of the analysis using the Random Forest (RF) algorithm with (F-score = 81%). This result outperformed the existing studies in the field of cyber threat detection and showed the importance of detecting cyber threats in social media posts. There is a need for more investigation in the field of multiclass classification to achieve more accurate results. In the future, this study suggests applying different data representations for the feature extraction other than TF-IDF such as Word2Vec, and adding a new phase for feature selection to select the optimum features subset to achieve higher accuracy of the detection process.Keywords
Cite This Article
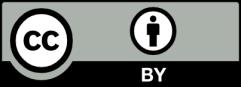
This work is licensed under a Creative Commons Attribution 4.0 International License , which permits unrestricted use, distribution, and reproduction in any medium, provided the original work is properly cited.