Open Access
ARTICLE
Modeling a Novel Hyper-Parameter Tuned Deep Learning Enabled Malaria Parasite Detection and Classification
1 Department of Computer Science & Engineering, Koneru Lakshmaiah Education Foundation, Vaddeswaram, Andhra Pradesh, India
2 Department of Artificial Intelligence and Data Science, KPR Institute of Engineering and Technology, Coimbatore, Tamil Nadu, 641407, India
* Corresponding Authors: Dinesh Kumar Anguraj. Email: ; S. V. Sudha. Email:
Computers, Materials & Continua 2023, 77(3), 3289-3304. https://doi.org/10.32604/cmc.2023.039515
Received 02 February 2023; Accepted 18 July 2023; Issue published 26 December 2023
Abstract
A theoretical methodology is suggested for finding the malaria parasites’ presence with the help of an intelligent hyper-parameter tuned Deep Learning (DL) based malaria parasite detection and classification (HPTDL-MPDC) in the smear images of human peripheral blood. Some existing approaches fail to predict the malaria parasitic features and reduce the prediction accuracy. The trained model initiated in the proposed system for classifying peripheral blood smear images into the non-parasite or parasite classes using the available online dataset. The Adagrad optimizer is stacked with the suggested pre-trained Deep Neural Network (DNN) with the help of the contrastive divergence method to pre-train. The features are extracted from the images in the proposed system to train the DNN for initializing the visible variables. The smear images show the concatenated feature to be utilized as the feature vector in the proposed system. Lastly, hyper-parameters are used to fine-tune DNN to calculate the class labels’ probability. The suggested system outperforms more modern methodologies with an accuracy of 91%, precision of 89%, recall of 93% and F1-score of 91%. The HPTDL-MPDC has the primary application in detecting the parasite of malaria in the smear images of human peripheral blood.Keywords
Cite This Article
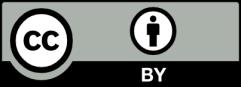
This work is licensed under a Creative Commons Attribution 4.0 International License , which permits unrestricted use, distribution, and reproduction in any medium, provided the original work is properly cited.