Open Access
ARTICLE
Real-Time Prediction Algorithm for Intelligent Edge Networks with Federated Learning-Based Modeling
1 Department of Software Convergence, Soonchunhyang University, Asan, 31538, Korea
2 Department of Telecommunication and Electronic Engineering, Royal University of Phnom Penh, Phnom Penh, 12156, Cambodia
3 Department of Computer Software Engineering, Soonchunhyang University, Asan, 31538, Korea
* Corresponding Author: Seokhoon Kim. Email:
Computers, Materials & Continua 2023, 77(2), 1967-1983. https://doi.org/10.32604/cmc.2023.045020
Received 14 August 2023; Accepted 27 September 2023; Issue published 29 November 2023
Abstract
Intelligent healthcare networks represent a significant component in digital applications, where the requirements hold within quality-of-service (QoS) reliability and safeguarding privacy. This paper addresses these requirements through the integration of enabler paradigms, including federated learning (FL), cloud/edge computing, software-defined/virtualized networking infrastructure, and converged prediction algorithms. The study focuses on achieving reliability and efficiency in real-time prediction models, which depend on the interaction flows and network topology. In response to these challenges, we introduce a modified version of federated logistic regression (FLR) that takes into account convergence latencies and the accuracy of the final FL model within healthcare networks. To establish the FLR framework for mission-critical healthcare applications, we provide a comprehensive workflow in this paper, introducing framework setup, iterative round communications, and model evaluation/deployment. Our optimization process delves into the formulation of loss functions and gradients within the domain of federated optimization, which concludes with the generation of service experience batches for model deployment. To assess the practicality of our approach, we conducted experiments using a hypertension prediction model with data sourced from the 2019 annual dataset (Version 2.0.1) of the Korea Medical Panel Survey. Performance metrics, including end-to-end execution delays, model drop/delivery ratios, and final model accuracies, are captured and compared between the proposed FLR framework and other baseline schemes. Our study offers an FLR framework setup for the enhancement of real-time prediction modeling within intelligent healthcare networks, addressing the critical demands of QoS reliability and privacy preservation.Keywords
Cite This Article
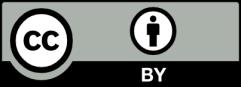
This work is licensed under a Creative Commons Attribution 4.0 International License , which permits unrestricted use, distribution, and reproduction in any medium, provided the original work is properly cited.