Open Access
ARTICLE
Flexible Global Aggregation and Dynamic Client Selection for Federated Learning in Internet of Vehicles
1 College of Information Technology, United Arab Emirates University, Al Ain, 17551, UAE
2 Department of Computing, School of EECS, National University of Sciences and Technology, Islamabad, 44000, Pakistan
3 College of Technological Innovation, Zayed University, Abu Dhabi, 144534, UAE
4 Department of Computer Science, The Superior University, Lahore, 54000, Pakistan
* Corresponding Author: Zouheir Trabelsi. Email:
(This article belongs to the Special Issue: Advanced Artificial Intelligence and Machine Learning Frameworks for Signal and Image Processing Applications)
Computers, Materials & Continua 2023, 77(2), 1739-1757. https://doi.org/10.32604/cmc.2023.043684
Received 10 July 2023; Accepted 18 September 2023; Issue published 29 November 2023
Abstract
Federated Learning (FL) enables collaborative and privacy-preserving training of machine learning models within the Internet of Vehicles (IoV) realm. While FL effectively tackles privacy concerns, it also imposes significant resource requirements. In traditional FL, trained models are transmitted to a central server for global aggregation, typically in the cloud. This approach often leads to network congestion and bandwidth limitations when numerous devices communicate with the same server. The need for Flexible Global Aggregation and Dynamic Client Selection in FL for the IoV arises from the inherent characteristics of IoV environments. These include diverse and distributed data sources, varying data quality, and limited communication resources. By employing dynamic client selection, we can prioritize relevant and high-quality data sources, enhancing model accuracy. To address this issue, we propose an FL framework that selects global aggregation nodes dynamically rather than a single fixed aggregator. Flexible global aggregation ensures efficient utilization of limited network resources while accommodating the dynamic nature of IoV data sources. This approach optimizes both model performance and resource allocation, making FL in IoV more effective and adaptable. The selection of the global aggregation node is based on workload and communication speed considerations. Additionally, our framework overcomes the constraints associated with network, computational, and energy resources in the IoV environment by implementing a client selection algorithm that dynamically adjusts participants according to predefined parameters. Our approach surpasses Federated Averaging (FedAvg) and Hierarchical FL (HFL) regarding energy consumption, delay, and accuracy, yielding superior results.Keywords
Cite This Article
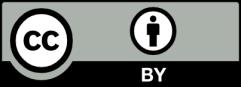