Open Access
ARTICLE
A Lightweight Road Scene Semantic Segmentation Algorithm
1 College of Automation, Guangxi University of Science and Technology, Liuzhou, 545000, China
2 Department of Artificial Intelligence and Manufacturing, Hechi University, Hechi, 547000, China
* Corresponding Author: Jiansheng Peng. Email:
(This article belongs to the Special Issue: Advanced Artificial Intelligence and Machine Learning Frameworks for Signal and Image Processing Applications)
Computers, Materials & Continua 2023, 77(2), 1929-1948. https://doi.org/10.32604/cmc.2023.043524
Received 04 July 2023; Accepted 20 September 2023; Issue published 29 November 2023
Abstract
In recent years, with the continuous deepening of smart city construction, there have been significant changes and improvements in the field of intelligent transportation. The semantic segmentation of road scenes has important practical significance in the fields of automatic driving, transportation planning, and intelligent transportation systems. However, the current mainstream lightweight semantic segmentation models in road scene segmentation face problems such as poor segmentation performance of small targets and insufficient refinement of segmentation edges. Therefore, this article proposes a lightweight semantic segmentation model based on the LiteSeg model improvement to address these issues. The model uses the lightweight backbone network MobileNet instead of the LiteSeg backbone network to reduce the network parameters and computation, and combines the Coordinate Attention (CA) mechanism to help the network capture long-distance dependencies. At the same time, by combining the dependencies of spatial information and channel information, the Spatial and Channel Network (SCNet) attention mechanism is proposed to improve the feature extraction ability of the model. Finally, a multi-scale transposed attention encoding (MTAE) module was proposed to obtain features of different resolutions and perform feature fusion. In this paper, the proposed model is verified on the Cityscapes dataset. The experimental results show that the addition of SCNet and MTAE modules increases the mean Intersection over Union (mIoU) of the original LiteSeg model by 4.69%. On this basis, the backbone network is replaced with MobileNet, and the CA model is added at the same time. At the cost of increasing the minimum model parameters and computing costs, the mIoU of the original LiteSeg model is increased by 2.46%. This article also compares the proposed model with some current lightweight semantic segmentation models, and experiments show that the comprehensive performance of the proposed model is the best, especially in achieving excellent results in small object segmentation. Finally, this article will conduct generalization testing on the KITTI dataset for the proposed model, and the experimental results show that the proposed algorithm has a certain degree of generalization.Keywords
Cite This Article
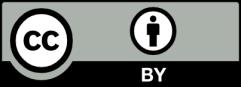
This work is licensed under a Creative Commons Attribution 4.0 International License , which permits unrestricted use, distribution, and reproduction in any medium, provided the original work is properly cited.