Open Access
ARTICLE
Gate-Attention and Dual-End Enhancement Mechanism for Multi-Label Text Classification
1 School of Computer Science and Technology, Hainan University, Haikou, 570228, China
2 Hainan Blockchain Technology Engineering Research Center, Hainan University, Haikou, 570228, China
3 School of Cyberspace Security, Hainan University, Haikou, 570228, China
4 Department of Computer Science, Texas Tech University, Lubbock, 79409, USA
* Corresponding Author: Xiaolong Chen. Email:
Computers, Materials & Continua 2023, 77(2), 1779-1793. https://doi.org/10.32604/cmc.2023.042980
Received 18 June 2023; Accepted 14 September 2023; Issue published 29 November 2023
Abstract
In the realm of Multi-Label Text Classification (MLTC), the dual challenges of extracting rich semantic features from text and discerning inter-label relationships have spurred innovative approaches. Many studies in semantic feature extraction have turned to external knowledge to augment the model’s grasp of textual content, often overlooking intrinsic textual cues such as label statistical features. In contrast, these endogenous insights naturally align with the classification task. In our paper, to complement this focus on intrinsic knowledge, we introduce a novel Gate-Attention mechanism. This mechanism adeptly integrates statistical features from the text itself into the semantic fabric, enhancing the model’s capacity to understand and represent the data. Additionally, to address the intricate task of mining label correlations, we propose a Dual-end enhancement mechanism. This mechanism effectively mitigates the challenges of information loss and erroneous transmission inherent in traditional long short term memory propagation. We conducted an extensive battery of experiments on the AAPD and RCV1-2 datasets. These experiments serve the dual purpose of confirming the efficacy of both the Gate-Attention mechanism and the Dual-end enhancement mechanism. Our final model unequivocally outperforms the baseline model, attesting to its robustness. These findings emphatically underscore the imperativeness of taking into account not just external knowledge but also the inherent intricacies of textual data when crafting potent MLTC models.Keywords
Cite This Article
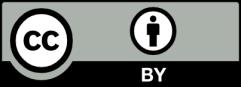