Open Access
ARTICLE
Swin-PAFF: A SAR Ship Detection Network with Contextual Cross-Information Fusion
School of Information Engineering, Shanghai Maritime University, Shanghai, 201306, China
* Corresponding Author: Yujun Zhang. Email:
Computers, Materials & Continua 2023, 77(2), 2657-2675. https://doi.org/10.32604/cmc.2023.042311
Received 25 May 2023; Accepted 15 August 2023; Issue published 29 November 2023
Abstract
Synthetic Aperture Radar (SAR) image target detection has widespread applications in both military and civil domains. However, SAR images pose challenges due to strong scattering, indistinct edge contours, multi-scale representation, sparsity, and severe background interference, which make the existing target detection methods in low accuracy. To address this issue, this paper proposes a multi-scale fusion framework (Swin-PAFF) for SAR target detection that utilizes the global context perception capability of the Transformer and the multi-layer feature fusion learning ability of the feature pyramid structure (FPN). Firstly, to tackle the issue of inadequate perceptual image context information in SAR target detection, we propose an end-to-end SAR target detection network with the Transformer structure as the backbone. Furthermore, we enhance the ability of the Swin Transformer to acquire contextual features and cross-information by incorporating a Swin-CC backbone network model that combines the Spatial Depthwise Pooling (SDP) module and the self-attentive mechanism. Finally, we design a cross-layer fusion neck module (PAFF) that better handles multi-scale variations and complex situations (such as sparsity, background interference, etc.). Our devised approach yields a noteworthy AP@0.5:0.95 performance of 91.3% when assessed on the HRSID dataset. The application of our proposed technique has resulted in a noteworthy advancement of 8% in the AP@0.5:0.95 scores on the HRSID dataset.Keywords
Cite This Article
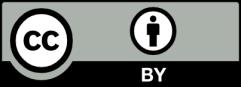
This work is licensed under a Creative Commons Attribution 4.0 International License , which permits unrestricted use, distribution, and reproduction in any medium, provided the original work is properly cited.