Open Access
ARTICLE
C2Net-YOLOv5: A Bidirectional Res2Net-Based Traffic Sign Detection Algorithm
1 Faculty of Information Technology, Beijing University of Technology, Beijing, 100124, China
2 School of Cyberspace Security, Beijing University of Posts and Telecommunications, Beijing, 100048, China
3 Fan Gongxiu Honors College, Beijing University of Technology, Beijing, 100124, China
* Corresponding Author: Yiqi Tian. Email:
(This article belongs to the Special Issue: Machine Vision Detection and Intelligent Recognition)
Computers, Materials & Continua 2023, 77(2), 1949-1965. https://doi.org/10.32604/cmc.2023.042224
Received 23 May 2023; Accepted 28 September 2023; Issue published 29 November 2023
Abstract
Rapid advancement of intelligent transportation systems (ITS) and autonomous driving (AD) have shown the importance of accurate and efficient detection of traffic signs. However, certain drawbacks, such as balancing accuracy and real-time performance, hinder the deployment of traffic sign detection algorithms in ITS and AD domains. In this study, a novel traffic sign detection algorithm was proposed based on the bidirectional Res2Net architecture to achieve an improved balance between accuracy and speed. An enhanced backbone network module, called C2Net, which uses an upgraded bidirectional Res2Net, was introduced to mitigate information loss in the feature extraction process and to achieve information complementarity. Furthermore, a squeeze-and-excitation attention mechanism was incorporated within the channel attention of the architecture to perform channel-level feature correction on the input feature map, which effectively retains valuable features while removing non-essential features. A series of ablation experiments were conducted to validate the efficacy of the proposed methodology. The performance was evaluated using two distinct datasets: the Tsinghua-Tencent 100K and the CSUST Chinese traffic sign detection benchmark 2021. On the TT100K dataset, the method achieves precision, recall, and Map0.5 scores of 83.3%, 79.3%, and 84.2%, respectively. Similarly, on the CCTSDB 2021 dataset, the method achieves precision, recall, and Map0.5 scores of 91.49%, 73.79%, and 81.03%, respectively. Experimental results revealed that the proposed method had superior performance compared to conventional models, which includes the faster region-based convolutional neural network, single shot multibox detector, and you only look once version 5.Keywords
Cite This Article
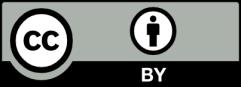
This work is licensed under a Creative Commons Attribution 4.0 International License , which permits unrestricted use, distribution, and reproduction in any medium, provided the original work is properly cited.