Open Access
ARTICLE
Optimized Deep Learning Approach for Efficient Diabetic Retinopathy Classification Combining VGG16-CNN
1 Department of Computer Science, the Higher Future Institute for Specialized Technological Studies, Obour, 11828, Egypt
2 Department of Electrical Engineering, Benha Faculty of Engineering, Benha University, Benha, 13511, Egypt
* Corresponding Author: Heba M. El-Hoseny. Email:
Computers, Materials & Continua 2023, 77(2), 1855-1872. https://doi.org/10.32604/cmc.2023.042107
Received 18 May 2023; Accepted 07 September 2023; Issue published 29 November 2023
Abstract
Diabetic retinopathy is a critical eye condition that, if not treated, can lead to vision loss. Traditional methods of diagnosing and treating the disease are time-consuming and expensive. However, machine learning and deep transfer learning (DTL) techniques have shown promise in medical applications, including detecting, classifying, and segmenting diabetic retinopathy. These advanced techniques offer higher accuracy and performance. Computer-Aided Diagnosis (CAD) is crucial in speeding up classification and providing accurate disease diagnoses. Overall, these technological advancements hold great potential for improving the management of diabetic retinopathy. The study’s objective was to differentiate between different classes of diabetes and verify the model’s capability to distinguish between these classes. The robustness of the model was evaluated using other metrics such as accuracy (ACC), precision (PRE), recall (REC), and area under the curve (AUC). In this particular study, the researchers utilized data cleansing techniques, transfer learning (TL), and convolutional neural network (CNN) methods to effectively identify and categorize the various diseases associated with diabetic retinopathy (DR). They employed the VGG-16CNN model, incorporating intelligent parameters that enhanced its robustness. The outcomes surpassed the results obtained by the auto enhancement (AE) filter, which had an ACC of over 98%. The manuscript provides visual aids such as graphs, tables, and techniques and frameworks to enhance understanding. This study highlights the significance of optimized deep TL in improving the metrics of the classification of the four separate classes of DR. The manuscript emphasizes the importance of using the VGG16CNN classification technique in this context.Keywords
Cite This Article
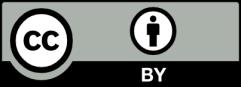
This work is licensed under a Creative Commons Attribution 4.0 International License , which permits unrestricted use, distribution, and reproduction in any medium, provided the original work is properly cited.