Open Access
ARTICLE
Electroencephalography (EEG) Based Neonatal Sleep Staging and Detection Using Various Classification Algorithms
1 Center for Intelligent Medical Electronics, Department of Electronic Engineering, Fudan University, Shanghai, 200433, China
2 Department of Biomedical Engineering, Riphah International University, Islamabad, 45320, Pakistan
* Corresponding Author: Saadullah Farooq Abbasi. Email:
Computers, Materials & Continua 2023, 77(2), 1759-1778. https://doi.org/10.32604/cmc.2023.041970
Received 12 May 2023; Accepted 11 September 2023; Issue published 29 November 2023
Abstract
Automatic sleep staging of neonates is essential for monitoring their brain development and maturity of the nervous system. EEG based neonatal sleep staging provides valuable information about an infant’s growth and health, but is challenging due to the unique characteristics of EEG and lack of standardized protocols. This study aims to develop and compare 18 machine learning models using Automated Machine Learning (autoML) technique for accurate and reliable multi-channel EEG-based neonatal sleep-wake classification. The study investigates autoML feasibility without extensive manual selection of features or hyperparameter tuning. The data is obtained from neonates at post-menstrual age 37 ± 05 weeks. 3525 30-s EEG segments from 19 infants are used to train and test the proposed models. There are twelve time and frequency domain features extracted from each channel. Each model receives the common features of nine channels as an input vector of size 108. Each model’s performance was evaluated based on a variety of evaluation metrics. The maximum mean accuracy of 84.78% and kappa of 69.63% has been obtained by the AutoML-based Random Forest estimator. This is the highest accuracy for EEG-based sleep-wake classification, until now. While, for the AutoML-based Adaboost Random Forest model, accuracy and kappa were 84.59% and 69.24%, respectively. High performance achieved in the proposed autoML-based approach can facilitate early identification and treatment of sleep-related issues in neonates.Keywords
Cite This Article
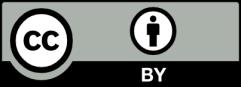