Open Access
ARTICLE
Automated Video Generation of Moving Digits from Text Using Deep Deconvolutional Generative Adversarial Network
1 National Engineering Research Center for E-Learning, Central China Normal University, Wuhan, 430079, China
2 Wollongong Joint Institute, Central China Normal University, Wuhan, 430079, China
* Corresponding Author: Xinguo Yu. Email:
(This article belongs to the Special Issue: Cognitive Computing and Systems in Education and Research)
Computers, Materials & Continua 2023, 77(2), 2359-2383. https://doi.org/10.32604/cmc.2023.041219
Received 14 April 2023; Accepted 19 June 2023; Issue published 29 November 2023
Abstract
Generating realistic and synthetic video from text is a highly challenging task due to the multitude of issues involved, including digit deformation, noise interference between frames, blurred output, and the need for temporal coherence across frames. In this paper, we propose a novel approach for generating coherent videos of moving digits from textual input using a Deep Deconvolutional Generative Adversarial Network (DD-GAN). The DD-GAN comprises a Deep Deconvolutional Neural Network (DDNN) as a Generator (G) and a modified Deep Convolutional Neural Network (DCNN) as a Discriminator (D) to ensure temporal coherence between adjacent frames. The proposed research involves several steps. First, the input text is fed into a Long Short Term Memory (LSTM) based text encoder and then smoothed using Conditioning Augmentation (CA) techniques to enhance the effectiveness of the Generator (G). Next, using a DDNN to generate video frames by incorporating enhanced text and random noise and modifying a DCNN to act as a Discriminator (D), effectively distinguishing between generated and real videos. This research evaluates the quality of the generated videos using standard metrics like Inception Score (IS), Fréchet Inception Distance (FID), Fréchet Inception Distance for video (FID2vid), and Generative Adversarial Metric (GAM), along with a human study based on realism, coherence, and relevance. By conducting experiments on Single-Digit Bouncing MNIST GIFs (SBMG), Two-Digit Bouncing MNIST GIFs (TBMG), and a custom dataset of essential mathematics videos with related text, this research demonstrates significant improvements in both metrics and human study results, confirming the effectiveness of DD-GAN. This research also took the exciting challenge of generating preschool math videos from text, handling complex structures, digits, and symbols, and achieving successful results. The proposed research demonstrates promising results for generating coherent videos from textual input.Keywords
Cite This Article
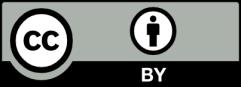
This work is licensed under a Creative Commons Attribution 4.0 International License , which permits unrestricted use, distribution, and reproduction in any medium, provided the original work is properly cited.