Open Access
ARTICLE
Diagnosis of Autism Spectrum Disorder by Imperialistic Competitive Algorithm and Logistic Regression Classifier
1 Department of Computer Science, College of Computer Engineering and Sciences, Prince Sattam bin Abdulaziz University, Al Kharj, 16274, Saudi Arabia
2 Department of Computer Science, College of Computer Science and Engineering, Taibah University, Madinah, 41411, Saudi Arabia
3 Department of Information Systems, College of Computer Engineering and Sciences, Prince Sattam bin Abdulaziz University, Al Kharj, 16274, Saudi Arabia
* Corresponding Author: Shabana R. Ziyad. Email:
Computers, Materials & Continua 2023, 77(2), 1515-1534. https://doi.org/10.32604/cmc.2023.040874
Received 02 April 2023; Accepted 25 July 2023; Issue published 29 November 2023
Abstract
Autism spectrum disorder (ASD), classified as a developmental disability, is now more common in children than ever. A drastic increase in the rate of autism spectrum disorder in children worldwide demands early detection of autism in children. Parents can seek professional help for a better prognosis of the child’s therapy when ASD is diagnosed under five years. This research study aims to develop an automated tool for diagnosing autism in children. The computer-aided diagnosis tool for ASD detection is designed and developed by a novel methodology that includes data acquisition, feature selection, and classification phases. The most deterministic features are selected from the self-acquired dataset by novel feature selection methods before classification. The Imperialistic competitive algorithm (ICA) based on empires conquering colonies performs feature selection in this study. The performance of Logistic Regression (LR), Decision tree, K-Nearest Neighbor (KNN), and Random Forest (RF) classifiers are experimentally studied in this research work. The experimental results prove that the Logistic regression classifier exhibits the highest accuracy for the self-acquired dataset. The ASD detection is evaluated experimentally with the Least Absolute Shrinkage and Selection Operator (LASSO) feature selection method and different classifiers. The Exploratory Data Analysis (EDA) phase has uncovered crucial facts about the data, like the correlation of the features in the dataset with the class variable.Keywords
Cite This Article
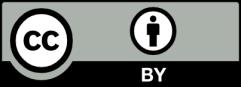
This work is licensed under a Creative Commons Attribution 4.0 International License , which permits unrestricted use, distribution, and reproduction in any medium, provided the original work is properly cited.