Open Access
ARTICLE
Towards Cache-Assisted Hierarchical Detection for Real-Time Health Data Monitoring in IoHT
1
School of Software Technology, Dalian University of Technology (DUT), Dalian, 116621, China
2
School of Software Technology, Key Laboratory for Ubiquitous Network and Service Software of Liaoning Province, Dalian,
116650, China
3
Department of Computer Engineering, King Saud University, Riyadh, 11543, Saudi Arabia
4
School of Software, Tsinghua University, Beijing, 100084, China
5
Department of Software Engineering, University of Science and Technology, Banu, 28100, Pakistan
6
Department of AI and Software, Gachon University, Seongnamsi, 13120, Korea
* Corresponding Authors: Muhammad Tahir. Email: ;
(This article belongs to the Special Issue: Big Data Analysis for Healthcare Applications)
Computers, Materials & Continua 2023, 77(2), 2529-2544. https://doi.org/10.32604/cmc.2023.042403
Received 29 May 2023; Accepted 13 October 2023; Issue published 29 November 2023
Abstract
Real-time health data monitoring is pivotal for bolstering road services’ safety, intelligence, and efficiency within the Internet of Health Things (IoHT) framework. Yet, delays in data retrieval can markedly hinder the efficacy of big data awareness detection systems. We advocate for a collaborative caching approach involving edge devices and cloud networks to combat this. This strategy is devised to streamline the data retrieval path, subsequently diminishing network strain. Crafting an adept cache processing scheme poses its own set of challenges, especially given the transient nature of monitoring data and the imperative for swift data transmission, intertwined with resource allocation tactics. This paper unveils a novel mobile healthcare solution that harnesses the power of our collaborative caching approach, facilitating nuanced health monitoring via edge devices. The system capitalizes on cloud computing for intricate health data analytics, especially in pinpointing health anomalies. Given the dynamic locational shifts and possible connection disruptions, we have architected a hierarchical detection system, particularly during crises. This system caches data efficiently and incorporates a detection utility to assess data freshness and potential lag in response times. Furthermore, we introduce the Cache-Assisted Real-Time Detection (CARD) model, crafted to optimize utility. Addressing the inherent complexity of the NP-hard CARD model, we have championed a greedy algorithm as a solution. Simulations reveal that our collaborative caching technique markedly elevates the Cache Hit Ratio (CHR) and data freshness, outshining its contemporaneous benchmark algorithms. The empirical results underscore the strength and efficiency of our innovative IoHT-based health monitoring solution. To encapsulate, this paper tackles the nuances of real-time health data monitoring in the IoHT landscape, presenting a joint edge-cloud caching strategy paired with a hierarchical detection system. Our methodology yields enhanced cache efficiency and data freshness. The corroborative numerical data accentuates the feasibility and relevance of our model, casting a beacon for the future trajectory of real-time health data monitoring systems.Keywords
Cite This Article
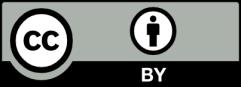
This work is licensed under a Creative Commons Attribution 4.0 International License , which permits unrestricted use, distribution, and reproduction in any medium, provided the original work is properly cited.