Open Access
ARTICLE
Optimizing Fully Convolutional Encoder-Decoder Network for Segmentation of Diabetic Eye Disease
1
Faculty of Information Technology, Beijing University of Technology, Beijing, 100124, China
2
College of Information Science Technology, Hainan Normal University, Haikou, 571158, China
* Corresponding Author: Guangmin Sun. Email:
(This article belongs to the Special Issue: Recent Advances in Ophthalmic Diseases Diagnosis using AI)
Computers, Materials & Continua 2023, 77(2), 2481-2504. https://doi.org/10.32604/cmc.2023.043239
Received 26 June 2023; Accepted 30 September 2023; Issue published 29 November 2023
Abstract
In the emerging field of image segmentation, Fully Convolutional Networks (FCNs) have recently become prominent. However, their effectiveness is intimately linked with the correct selection and fine-tuning of hyperparameters, which can often be a cumbersome manual task. The main aim of this study is to propose a more efficient, less labour-intensive approach to hyperparameter optimization in FCNs for segmenting fundus images. To this end, our research introduces a hyperparameter-optimized Fully Convolutional Encoder-Decoder Network (FCEDN). The optimization is handled by a novel Genetic Grey Wolf Optimization (G-GWO) algorithm. This algorithm employs the Genetic Algorithm (GA) to generate a diverse set of initial positions. It leverages Grey Wolf Optimization (GWO) to fine-tune these positions within the discrete search space. Testing on the Indian Diabetic Retinopathy Image Dataset (IDRiD), Diabetic Retinopathy, Hypertension, Age-related macular degeneration and Glacuoma ImageS (DR-HAGIS), and Ocular Disease Intelligent Recognition (ODIR) datasets showed that the G-GWO method outperformed four other variants of GWO, GA, and PSO-based hyperparameter optimization techniques. The proposed model achieved impressive segmentation results, with accuracy rates of 98.5% for IDRiD, 98.7% for DR-HAGIS, and 98.4%, 98.8%, and 98.5% for different sub-datasets within ODIR. These results suggest that the proposed hyperparameter-optimized FCEDN model, driven by the G-GWO algorithm, is more efficient than recent deep-learning models for image segmentation tasks. It thereby presents the potential for increased automation and accuracy in the segmentation of fundus images, mitigating the need for extensive manual hyperparameter adjustments.Keywords
Cite This Article
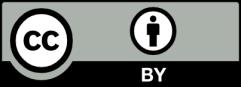