Open Access
ARTICLE
Detecting and Mitigating DDOS Attacks in SDNs Using Deep Neural Network
1
Department of Information Technology, The University of Haripur, Haripur, 22060, Pakistan
2
Faculty of Computing and Informatics, University Malaysia Sabah, Sabah, 88400, Malaysia
3
Department of Environmental Sciences, COMSATS University Abbottabad Campus, Abbottabad, 22010, Pakistan
4
Department of Chemistry, COMSATS University Abbottabad Campus, Abbottabad, 22010, Pakistan
* Corresponding Author: Abdullah Gani. Email:
Computers, Materials & Continua 2023, 77(2), 2157-2178. https://doi.org/10.32604/cmc.2023.026952
Received 08 January 2022; Accepted 22 April 2022; Issue published 29 November 2023
Abstract
Distributed denial of service (DDoS) attack is the most common attack that obstructs a network and makes it unavailable for a legitimate user. We proposed a deep neural network (DNN) model for the detection of DDoS attacks in the Software-Defined Networking (SDN) paradigm. SDN centralizes the control plane and separates it from the data plane. It simplifies a network and eliminates vendor specification of a device. Because of this open nature and centralized control, SDN can easily become a victim of DDoS attacks. We proposed a supervised Developed Deep Neural Network (DDNN) model that can classify the DDoS attack traffic and legitimate traffic. Our Developed Deep Neural Network (DDNN) model takes a large number of feature values as compared to previously proposed Machine Learning (ML) models. The proposed DNN model scans the data to find the correlated features and delivers high-quality results. The model enhances the security of SDN and has better accuracy as compared to previously proposed models. We choose the latest state-of-the-art dataset which consists of many novel attacks and overcomes all the shortcomings and limitations of the existing datasets. Our model results in a high accuracy rate of 99.76% with a low false-positive rate and 0.065% low loss rate. The accuracy increases to 99.80% as we increase the number of epochs to 100 rounds. Our proposed model classifies anomalous and normal traffic more accurately as compared to the previously proposed models. It can handle a huge amount of structured and unstructured data and can easily solve complex problems.Keywords
Cite This Article
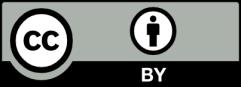