Open Access
ARTICLE
VGWO: Variant Grey Wolf Optimizer with High Accuracy and Low Time Complexity
1
School of Information Science and Engineering, Hunan Institute of Science and Technology, Yueyang, 414006, China
2
Department of Computer and Information Science, Linköping University, Linköping, 58183, Sweden
3
Department of Safety and Quality, Hunan Construction Investment Group, Changsha, 410004, China
* Corresponding Author: Bo Fan. Email:
Computers, Materials & Continua 2023, 77(2), 1617-1644. https://doi.org/10.32604/cmc.2023.041973
Received 13 May 2023; Accepted 07 September 2023; Issue published 29 November 2023
Abstract
The grey wolf optimizer (GWO) is a swarm-based intelligence optimization algorithm by simulating the steps of searching, encircling, and attacking prey in the process of wolf hunting. Along with its advantages of simple principle and few parameters setting, GWO bears drawbacks such as low solution accuracy and slow convergence speed. A few recent advanced GWOs are proposed to try to overcome these disadvantages. However, they are either difficult to apply to large-scale problems due to high time complexity or easily lead to early convergence. To solve the abovementioned issues, a high-accuracy variable grey wolf optimizer (VGWO) with low time complexity is proposed in this study. VGWO first uses the symmetrical wolf strategy to generate an initial population of individuals to lay the foundation for the global seek of the algorithm, and then inspired by the simulated annealing algorithm and the differential evolution algorithm, a mutation operation for generating a new mutant individual is performed on three wolves which are randomly selected in the current wolf individuals while after each iteration. A vectorized Manhattan distance calculation method is specifically designed to evaluate the probability of selecting the mutant individual based on its status in the current wolf population for the purpose of dynamically balancing global search and fast convergence capability of VGWO. A series of experiments are conducted on 19 benchmark functions from CEC2014 and CEC2020 and three real-world engineering cases. For 19 benchmark functions, VGWO’s optimization results place first in 80% of comparisons to the state-of-art GWOs and the CEC2020 competition winner. A further evaluation based on the Friedman test, VGWO also outperforms all other algorithms statistically in terms of robustness with a better average ranking value.Keywords
Cite This Article
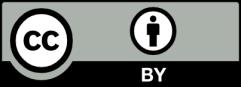
This work is licensed under a Creative Commons Attribution 4.0 International License , which permits unrestricted use, distribution, and reproduction in any medium, provided the original work is properly cited.