Open Access
ARTICLE
Chinese Cyber Threat Intelligence Named Entity Recognition via RoBERTa-wwm-RDCNN-CRF
1
School of Information Network Security, People’s Public Security University of China, Beijing, 100038, China
2
Key Laboratory of Safety Precautions and Risk Assessment, Ministry of Public Security, Beijing, 102623, China
* Corresponding Author: Jian Gao. Email:
Computers, Materials & Continua 2023, 77(1), 299-323. https://doi.org/10.32604/cmc.2023.042090
Received 18 May 2023; Accepted 15 September 2023; Issue published 31 October 2023
Abstract
In recent years, cyber attacks have been intensifying and causing great harm to individuals, companies, and countries. The mining of cyber threat intelligence (CTI) can facilitate intelligence integration and serve well in combating cyber attacks. Named Entity Recognition (NER), as a crucial component of text mining, can structure complex CTI text and aid cybersecurity professionals in effectively countering threats. However, current CTI NER research has mainly focused on studying English CTI. In the limited studies conducted on Chinese text, existing models have shown poor performance. To fully utilize the power of Chinese pre-trained language models (PLMs) and conquer the problem of lengthy infrequent English words mixing in the Chinese CTIs, we propose a residual dilated convolutional neural network (RDCNN) with a conditional random field (CRF) based on a robustly optimized bidirectional encoder representation from transformers pre-training approach with whole word masking (RoBERTa-wwm), abbreviated as RoBERTa-wwm-RDCNN-CRF. We are the first to experiment on the relevant open source dataset and achieve an F1-score of 82.35%, which exceeds the common baseline model bidirectional encoder representation from transformers (BERT)-bidirectional long short-term memory (BiLSTM)-CRF in this field by about 19.52% and exceeds the current state-of-the-art model, BERT-RDCNN-CRF, by about 3.53%. In addition, we conducted an ablation study on the encoder part of the model to verify the effectiveness of the proposed model and an in-depth investigation of the PLMs and encoder part of the model to verify the effectiveness of the proposed model. The RoBERTa-wwm-RDCNN-CRF model, the shared pre-processing, and augmentation methods can serve the subsequent fundamental tasks such as cybersecurity information extraction and knowledge graph construction, contributing to important applications in downstream tasks such as intrusion detection and advanced persistent threat (APT) attack detection.Keywords
Cite This Article
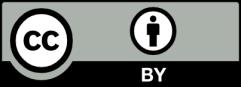