Open Access
ARTICLE
Soil NOx Emission Prediction via Recurrent Neural Networks
1 Department of Mechanical Engineering, Iowa Technology Institute, University of Iowa, Iowa City, IA 52242, USA
2 Department of Chemical and Biochemical Engineering, Iowa Technology Institute, University of Iowa, Iowa City, IA 52242, USA
* Corresponding Author: Shaoping Xiao. Email:
Computers, Materials & Continua 2023, 77(1), 285-297. https://doi.org/10.32604/cmc.2023.044366
Received 28 July 2023; Accepted 15 September 2023; Issue published 31 October 2023
Abstract
This paper presents designing sequence-to-sequence recurrent neural network (RNN) architectures for a novel study to predict soil NOx emissions, driven by the imperative of understanding and mitigating environmental impact. The study utilizes data collected by the Environmental Protection Agency (EPA) to develop two distinct RNN predictive models: one built upon the long-short term memory (LSTM) and the other utilizing the gated recurrent unit (GRU). These models are fed with a combination of historical and anticipated air temperature, air moisture, and NOx emissions as inputs to forecast future NOx emissions. Both LSTM and GRU models can capture the intricate pulse patterns inherent in soil NOx emissions. Notably, the GRU model emerges as the superior performer, surpassing the LSTM model in predictive accuracy while demonstrating efficiency by necessitating less training time. Intriguingly, the investigation into varying input features reveals that relying solely on past NOx emissions as input yields satisfactory performance, highlighting the dominant influence of this factor. The study also delves into the impact of altering input series lengths and training data sizes, yielding insights into optimal configurations for enhanced model performance. Importantly, the findings promise to advance our grasp of soil NOx emission dynamics, with implications for environmental management strategies. Looking ahead, the anticipated availability of additional measurements is poised to bolster machine-learning model efficacy. Furthermore, the future study will explore physical-based RNNs, a promising avenue for deeper insights into soil NOx emission prediction.Keywords
Cite This Article
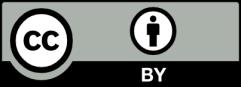
This work is licensed under a Creative Commons Attribution 4.0 International License , which permits unrestricted use, distribution, and reproduction in any medium, provided the original work is properly cited.