Open Access
ARTICLE
Traffic Sign Recognition for Autonomous Vehicle Using Optimized YOLOv7 and Convolutional Block Attention Module
1 School of Computer Science and Engineering, VIT-AP University, Andhra Pradesh, 522237, India
2 School of Creative Technology, University of Bolton, Manchester, BL3 5AB, UK
* Corresponding Author: P. Kuppusamy. Email:
Computers, Materials & Continua 2023, 77(1), 445-466. https://doi.org/10.32604/cmc.2023.042675
Received 07 June 2023; Accepted 16 August 2023; Issue published 31 October 2023
Abstract
The infrastructure and construction of roads are crucial for the economic and social development of a region, but traffic-related challenges like accidents and congestion persist. Artificial Intelligence (AI) and Machine Learning (ML) have been used in road infrastructure and construction, particularly with the Internet of Things (IoT) devices. Object detection in Computer Vision also plays a key role in improving road infrastructure and addressing traffic-related problems. This study aims to use You Only Look Once version 7 (YOLOv7), Convolutional Block Attention Module (CBAM), the most optimized object-detection algorithm, to detect and identify traffic signs, and analyze effective combinations of adaptive optimizers like Adaptive Moment estimation (Adam), Root Mean Squared Propagation (RMSprop) and Stochastic Gradient Descent (SGD) with the YOLOv7. Using a portion of German traffic signs for training, the study investigates the feasibility of adopting smaller datasets while maintaining high accuracy. The model proposed in this study not only improves traffic safety by detecting traffic signs but also has the potential to contribute to the rapid development of autonomous vehicle systems. The study results showed an impressive accuracy of 99.7% when using a batch size of 8 and the Adam optimizer. This high level of accuracy demonstrates the effectiveness of the proposed model for the image classification task of traffic sign recognition.Keywords
Cite This Article
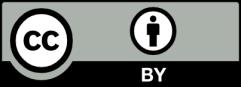
This work is licensed under a Creative Commons Attribution 4.0 International License , which permits unrestricted use, distribution, and reproduction in any medium, provided the original work is properly cited.