Open Access
ARTICLE
Convolution-Based Heterogeneous Activation Facility for Effective Machine Learning of ECG Signals
School of Electronics Engineering, Vellore Institute of Technology, Chennai, 600127, India
* Corresponding Author: Sathiya Narayanan. Email:
Computers, Materials & Continua 2023, 77(1), 25-45. https://doi.org/10.32604/cmc.2023.042590
Received 05 June 2023; Accepted 12 August 2023; Issue published 31 October 2023
Abstract
Machine Learning (ML) and Deep Learning (DL) technologies are revolutionizing the medical domain, especially with Electrocardiogram (ECG), by providing new tools and techniques for diagnosing, treating, and preventing diseases. However, DL architectures are computationally more demanding. In recent years, researchers have focused on combining the computationally less intensive portion of the DL architectures with ML approaches, say for example, combining the convolutional layer blocks of Convolution Neural Networks (CNNs) into ML algorithms such as Extreme Gradient Boosting (XGBoost) and K-Nearest Neighbor (KNN) resulting in CNN-XGBoost and CNN-KNN, respectively. However, these approaches are homogenous in the sense that they use a fixed Activation Function (AFs) in the sequence of convolution and pooling layers, thereby limiting the ability to capture unique features. Since various AFs are readily available and each could capture unique features, we propose a Convolution-based Heterogeneous Activation Facility (CHAF) which uses multiple AFs in the convolution layer blocks, one for each block, with a motivation of extracting features in a better manner to improve the accuracy. The proposed CHAF approach is validated on PTB and shown to outperform the homogeneous approaches such as CNN-KNN and CNN-XGBoost. For PTB dataset, proposed CHAF-KNN has an accuracy of 99.55% and an F1 score of 99.68% in just 0.008 s, outperforming the state-of-the-art CNN-XGBoost which has an accuracy of 99.38% and an F1 score of 99.32% in 1.23 s. To validate the generality of the proposed CHAF, experiments were repeated on MIT-BIH dataset, and the proposed CHAF-KNN is shown to outperform CNN-KNN and CNN-XGBoost.Keywords
Cite This Article
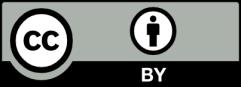
This work is licensed under a Creative Commons Attribution 4.0 International License , which permits unrestricted use, distribution, and reproduction in any medium, provided the original work is properly cited.