Open Access
ARTICLE
A Robust Conformer-Based Speech Recognition Model for Mandarin Air Traffic Control
1 College of Air Traffic Management, Civil Aviation Flight University of China, Deyang, 618307, China
2 East China Air Traffic Management Bureau, Xiamen Air Traffic Management Station, Xiamen, 361015, China
* Corresponding Author: Weijun Pan. Email:
(This article belongs to the Special Issue: Optimization for Artificial Intelligence Application)
Computers, Materials & Continua 2023, 77(1), 911-940. https://doi.org/10.32604/cmc.2023.041772
Received 05 May 2023; Accepted 10 August 2023; Issue published 31 October 2023
Abstract
This study aims to address the deviation in downstream tasks caused by inaccurate recognition results when applying Automatic Speech Recognition (ASR) technology in the Air Traffic Control (ATC) field. This paper presents a novel cascaded model architecture, namely Conformer-CTC/Attention-T5 (CCAT), to build a highly accurate and robust ATC speech recognition model. To tackle the challenges posed by noise and fast speech rate in ATC, the Conformer model is employed to extract robust and discriminative speech representations from raw waveforms. On the decoding side, the Attention mechanism is integrated to facilitate precise alignment between input features and output characters. The Text-To-Text Transfer Transformer (T5) language model is also introduced to handle particular pronunciations and code-mixing issues, providing more accurate and concise textual output for downstream tasks. To enhance the model’s robustness, transfer learning and data augmentation techniques are utilized in the training strategy. The model’s performance is optimized by performing hyperparameter tunings, such as adjusting the number of attention heads, encoder layers, and the weights of the loss function. The experimental results demonstrate the significant contributions of data augmentation, hyperparameter tuning, and error correction models to the overall model performance. On the Our ATC Corpus dataset, the proposed model achieves a Character Error Rate (CER) of 3.44%, representing a 3.64% improvement compared to the baseline model. Moreover, the effectiveness of the proposed model is validated on two publicly available datasets. On the AISHELL-1 dataset, the CCAT model achieves a CER of 3.42%, showcasing a 1.23% improvement over the baseline model. Similarly, on the LibriSpeech dataset, the CCAT model achieves a Word Error Rate (WER) of 5.27%, demonstrating a performance improvement of 7.67% compared to the baseline model. Additionally, this paper proposes an evaluation criterion for assessing the robustness of ATC speech recognition systems. In robustness evaluation experiments based on this criterion, the proposed model demonstrates a performance improvement of 22% compared to the baseline model.
Keywords
Cite This Article
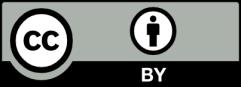
This work is licensed under a Creative Commons Attribution 4.0 International License , which permits unrestricted use, distribution, and reproduction in any medium, provided the original work is properly cited.