Open Access
ARTICLE
Recognizing Breast Cancer Using Edge-Weighted Texture Features of Histopathology Images
1 Department of Computer Science and Information Technology, Superior University, Lahore, 54000, Pakistan
2MLC Lab, Maharban House, House # 209, Zafar Colony, Okara, 56300, Pakistan
3 Information Technology Services, University of Okara, Okara, 56300, Pakistan
4 Department of CS&SE, International Islamic University, Islamabad, 44000, Pakistan
5 Department of Information Systems, College of Computer and Information Sciences, Princess Nourah bint Abdulrahman University, Riyadh, 11671, Saudi Arabia
6 Department of Computer Science, University of Okara, Okara, 56300, Pakistan
7 Department of Statistics and Computer Science, University of Veterinary and Animal Sciences, Lahore, Punjab, 54000, Pakistan
8 School of Biochemistry and Biotechnology, University of the Punjab, Lahore, 54000, Pakistan
9 Department of Computer Science, Bahria University, Lahore Campus, Lahore, 54600, Pakistan
* Corresponding Author: Nadeem Sarwar. Email:
(This article belongs to the Special Issue: Big Data Analysis for Healthcare Applications)
Computers, Materials & Continua 2023, 77(1), 1081-1101. https://doi.org/10.32604/cmc.2023.041558
Received 27 April 2023; Accepted 12 August 2023; Issue published 31 October 2023
Abstract
Around one in eight women will be diagnosed with breast cancer at some time. Improved patient outcomes necessitate both early detection and an accurate diagnosis. Histological images are routinely utilized in the process of diagnosing breast cancer. Methods proposed in recent research only focus on classifying breast cancer on specific magnification levels. No study has focused on using a combined dataset with multiple magnification levels to classify breast cancer. A strategy for detecting breast cancer is provided in the context of this investigation. Histopathology image texture data is used with the wavelet transform in this technique. The proposed method comprises converting histopathological images from Red Green Blue (RGB) to Chrominance of Blue and Chrominance of Red (YCBCR), utilizing a wavelet transform to extract texture information, and classifying the images with Extreme Gradient Boosting (XGBOOST). Furthermore, SMOTE has been used for resampling as the dataset has imbalanced samples. The suggested method is evaluated using 10-fold cross-validation and achieves an accuracy of 99.27% on the BreakHis 1.0 40X dataset, 98.95% on the BreakHis 1.0 100X dataset, 98.92% on the BreakHis 1.0 200X dataset, 98.78% on the BreakHis 1.0 400X dataset, and 98.80% on the combined dataset. The findings of this study imply that improved breast cancer detection rates and patient outcomes can be achieved by combining wavelet transformation with textural signals to detect breast cancer in histopathology images.Keywords
Cite This Article
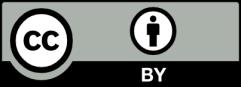
This work is licensed under a Creative Commons Attribution 4.0 International License , which permits unrestricted use, distribution, and reproduction in any medium, provided the original work is properly cited.