Open Access
ARTICLE
An Efficient Stacked Ensemble Model for Heart Disease Detection and Classification
1 Department of Computer Science, COMSATS University, Islamabad, Pakistan
2 College of Computer Studies, De La Salle University, Manila, 1004, Philippines
3 Faculty of Information and Communication Studies, University of the Philippines Open University, Los Banos, 4031, Philippines
4 College of Computer Engineering and Sciences, Prince Sattam bin Abdulaziz University, Alkharj, Saudi Arabia
5 Department of Computer Engineering and Networks, College of Computer and Information Sciences, Jouf University, Sakaka, 72388, Saudi Arabia
6 School of Electrical and Computer Engineering, Yeosu Campus, Chonnam National University, Yeosu-si, 59626, Korea
* Corresponding Author: Tai-hoon Kim. Email:
Computers, Materials & Continua 2023, 77(1), 665-680. https://doi.org/10.32604/cmc.2023.041031
Received 08 April 2023; Accepted 19 June 2023; Issue published 31 October 2023
Abstract
Cardiac disease is a chronic condition that impairs the heart’s functionality. It includes conditions such as coronary artery disease, heart failure, arrhythmias, and valvular heart disease. These conditions can lead to serious complications and even be life-threatening if not detected and managed in time. Researchers have utilized Machine Learning (ML) and Deep Learning (DL) to identify heart abnormalities swiftly and consistently. Various approaches have been applied to predict and treat heart disease utilizing ML and DL. This paper proposes a Machine and Deep Learning-based Stacked Model (MDLSM) to predict heart disease accurately. ML approaches such as eXtreme Gradient Boosting (XGB), Random Forest (RF), Naive Bayes (NB), Decision Tree (DT), and K-Nearest Neighbor (KNN), along with two DL models: Deep Neural Network (DNN) and Fine Tuned Deep Neural Network (FT-DNN) are used to detect heart disease. These models rely on electronic medical data that increases the likelihood of correctly identifying and diagnosing heart disease. Well-known evaluation measures (i.e., accuracy, precision, recall, F1-score, confusion matrix, and area under the Receiver Operating Characteristic (ROC) curve) are employed to check the efficacy of the proposed approach. Results reveal that the MDLSM achieves 94.14% prediction accuracy, which is 8.30% better than the results from the baseline experiments recommending our proposed approach for identifying and diagnosing heart disease.Keywords
Cite This Article
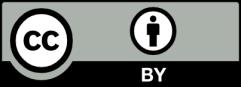
This work is licensed under a Creative Commons Attribution 4.0 International License , which permits unrestricted use, distribution, and reproduction in any medium, provided the original work is properly cited.