Open Access
ARTICLE
Multi-Modal Military Event Extraction Based on Knowledge Fusion
School of Computer Science, Liaocheng University, Liaocheng, 252059, China
* Corresponding Author: Yangli Jia. Email:
Computers, Materials & Continua 2023, 77(1), 97-114. https://doi.org/10.32604/cmc.2023.040751
Received 29 March 2023; Accepted 29 August 2023; Issue published 31 October 2023
Abstract
Event extraction stands as a significant endeavor within the realm of information extraction, aspiring to automatically extract structured event information from vast volumes of unstructured text. Extracting event elements from multi-modal data remains a challenging task due to the presence of a large number of images and overlapping event elements in the data. Although researchers have proposed various methods to accomplish this task, most existing event extraction models cannot address these challenges because they are only applicable to text scenarios. To solve the above issues, this paper proposes a multi-modal event extraction method based on knowledge fusion. Specifically, for event-type recognition, we use a meticulous pipeline approach that integrates multiple pre-trained models. This approach enables a more comprehensive capture of the multidimensional event semantic features present in military texts, thereby enhancing the interconnectedness of information between trigger words and events. For event element extraction, we propose a method for constructing a priori templates that combine event types with corresponding trigger words. This approach facilitates the acquisition of fine-grained input samples containing event trigger words, thus enabling the model to understand the semantic relationships between elements in greater depth. Furthermore, a fusion method for spatial mapping of textual event elements and image elements is proposed to reduce the category number overload and effectively achieve multi-modal knowledge fusion. The experimental results based on the CCKS 2022 dataset show that our method has achieved competitive results, with a comprehensive evaluation value F1-score of 53.4% for the model. These results validate the effectiveness of our method in extracting event elements from multi-modal data.Keywords
Cite This Article
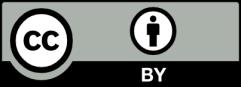