Open Access
ARTICLE
A Smart Heart Disease Diagnostic System Using Deep Vanilla LSTM
1 Department of Computer Science, COMSATS University Islamabad, Attock Campus, Attock, Pakistan
2 Department of Computer Science, COMSATS University Islamabad, Islamabad, Pakistan
3 Department of Computer Science, UET Taxila, Taxila, Pakistan
4 Department of AI and Big Data, Soonchunhyang University, Asan, Korea
5 Department of Industrial Security, Chung-Ang University, Seoul, 06974, Korea
* Corresponding Author: Seungmin Rho. Email:
Computers, Materials & Continua 2023, 77(1), 1251-1279. https://doi.org/10.32604/cmc.2023.040329
Received 14 March 2023; Accepted 19 June 2023; Issue published 31 October 2023
Abstract
Effective smart healthcare frameworks contain novel and emerging solutions for remote disease diagnostics, which aid in the prevention of several diseases including heart-related abnormalities. In this context, regular monitoring of cardiac patients through smart healthcare systems based on Electrocardiogram (ECG) signals has the potential to save many lives. In existing studies, several heart disease diagnostic systems are proposed by employing different state-of-the-art methods, however, improving such methods is always an intriguing area of research. Hence, in this research, a smart healthcare system is proposed for the diagnosis of heart disease using ECG signals. The proposed framework extracts both linear and time-series information on the ECG signals and fuses them into a single framework concurrently. The linear characteristics of ECG signals are extracted by convolution layers followed by Gaussian Error Linear Units (GeLu) and time series characteristics of ECG beats are extracted by Vanilla Long Short-Term Memory Networks (LSTM). Following on, the feature reduction of linear information is done with the help of ID Generalized Gated Pooling (GGP). In addition, data misbalancing issues are also addressed with the help of the Synthetic Minority Oversampling Technique (SMOTE). The performance assessment of the proposed model is done over the two publicly available datasets named MIT-BIH arrhythmia database (MITDB) and PTB Diagnostic ECG database (PTBDB). The proposed framework achieves an average accuracy performance of 99.14% along with a 95% recall value.Keywords
Cite This Article
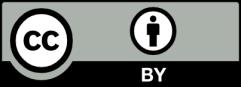
This work is licensed under a Creative Commons Attribution 4.0 International License , which permits unrestricted use, distribution, and reproduction in any medium, provided the original work is properly cited.