Open Access
ARTICLE
SmokerViT: A Transformer-Based Method for Smoker Recognition
1 College of Mathematics and Computer Science, Zhejiang Normal University, Jinhua, 321004, China
2 School of Electronics Engineering, Beijing University of Posts and Telecommunications, Beijing, 100876, China
3 Department of Electrical Engineering and Computer Science, Khalifa University of Science and Technology, Abu Dhabi, 127788, United Arab Emirates
4 Key Laboratory of Intelligent Education of Zhejiang Province, Zhejiang Normal University, Jinhua, 321004, China
* Corresponding Author: Zhonglong Zheng. Email:
Computers, Materials & Continua 2023, 77(1), 403-424. https://doi.org/10.32604/cmc.2023.040251
Received 10 March 2023; Accepted 01 July 2023; Issue published 31 October 2023
Abstract
Smoking has an economic and environmental impact on society due to the toxic substances it emits. Convolutional Neural Networks (CNNs) need help describing low-level features and can miss important information. Moreover, accurate smoker detection is vital with minimum false alarms. To answer the issue, the researchers of this paper have turned to a self-attention mechanism inspired by the ViT, which has displayed state-of-the-art performance in the classification task. To effectively enforce the smoking prohibition in non-smoking locations, this work presents a Vision Transformer-inspired model called SmokerViT for detecting smokers. Moreover, this research utilizes a locally curated dataset of 1120 images evenly distributed among the two classes (Smoking and NotSmoking). Further, this research performs augmentations on the smoker detection dataset to have many images with various representations to overcome the dataset size limitation. Unlike convolutional operations used in most existing works, the proposed SmokerViT model employs a self-attention mechanism in the Transformer block, making it suitable for the smoker classification problem. Besides, this work integrates the multi-layer perceptron head block in the SmokerViT model, which contains dense layers with rectified linear activation and linear kernel regularizer with L2 for the recognition task. This work presents an exhaustive analysis to prove the efficiency of the proposed SmokerViT model. The performance of the proposed SmokerViT performance is evaluated and compared with the existing methods, where it achieves an overall classification accuracy of 97.77%, with 98.21% recall and 97.35% precision, outperforming the state-of-the-art deep learning models, including convolutional neural networks (CNNs) and other vision transformer-based models.Keywords
Cite This Article
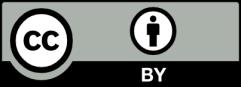