Open Access
ARTICLE
Solar Power Plant Network Packet-Based Anomaly Detection System for Cybersecurity
1 Department of Information Security, Gachon University, Seongnam, 13120, Korea
2 Police Science Institute, Korean National Police University, Asan, 31539, Korea
3 Department of Computer Engineering, Gachon University, Seongnam, 13120, Korea
* Corresponding Author: Jung Taek Seo. Email:
(This article belongs to the Special Issue: Advances in Information Security Application)
Computers, Materials & Continua 2023, 77(1), 757-779. https://doi.org/10.32604/cmc.2023.039461
Received 31 January 2023; Accepted 21 June 2023; Issue published 31 October 2023
Abstract
As energy-related problems continue to emerge, the need for stable energy supplies and issues regarding both environmental and safety require urgent consideration. Renewable energy is becoming increasingly important, with solar power accounting for the most significant proportion of renewables. As the scale and importance of solar energy have increased, cyber threats against solar power plants have also increased. So, we need an anomaly detection system that effectively detects cyber threats to solar power plants. However, as mentioned earlier, the existing solar power plant anomaly detection system monitors only operating information such as power generation, making it difficult to detect cyberattacks. To address this issue, in this paper, we propose a network packet-based anomaly detection system for the Programmable Logic Controller (PLC) of the inverter, an essential system of photovoltaic plants, to detect cyber threats. Cyberattacks and vulnerabilities in solar power plants were analyzed to identify cyber threats in solar power plants. The analysis shows that Denial of Service (DoS) and Man-in-the-Middle (MitM) attacks are primarily carried out on inverters, aiming to disrupt solar plant operations. To develop an anomaly detection system, we performed preprocessing, such as correlation analysis and normalization for PLC network packets data and trained various machine learning-based classification models on such data. The Random Forest model showed the best performance with an accuracy of 97.36%. The proposed system can detect anomalies based on network packets, identify potential cyber threats that cannot be identified by the anomaly detection system currently in use in solar power plants, and enhance the security of solar plants.Keywords
Cite This Article
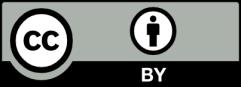