Open Access
ARTICLE
Deep Learning-Based Model for Detection of Brinjal Weed in the Era of Precision Agriculture
1 Department of Computer Science and Engineering, Institute of Technology, Nirma University, Ahmedabad, 382481, India
2 Software Engineering Department, College of Computer and Information Sciences, King Saud University, Riyadh, 12372, Saudi Arabia
3 Computer Science Department, Community College, King Saud University, Riyadh, 11437, Saudi Arabia
4 Centre for Inter-Disciplinary Research and Innovation, University of Petroleum and Energy Studies, Dehradun, 248001, India
5 Doctoral School, University Politehnica of Bucharest, Bucharest, 060042, Romania
6 National Research and Development Institute for Cryogenic and Isotopic Technologies-ICSI Rm, Valcea, Ramnicu Valcea, 240050, Romania
7 Power Engineering Department, Gheorghe Asachi Technical University of Iasi, Iasi, 700050, Romania
* Corresponding Authors: Sudeep Tanwar. Email: ; Maria Simona Raboaca. Email:
Computers, Materials & Continua 2023, 77(1), 1281-1301. https://doi.org/10.32604/cmc.2023.038796
Received 29 December 2022; Accepted 10 April 2023; Issue published 31 October 2023
Abstract
The overgrowth of weeds growing along with the primary crop in the fields reduces crop production. Conventional solutions like hand weeding are labor-intensive, costly, and time-consuming; farmers have used herbicides. The application of herbicide is effective but causes environmental and health concerns. Hence, Precision Agriculture (PA) suggests the variable spraying of herbicides so that herbicide chemicals do not affect the primary plants. Motivated by the gap above, we proposed a Deep Learning (DL) based model for detecting Eggplant (Brinjal) weed in this paper. The key objective of this study is to detect plant and non-plant (weed) parts from crop images. With the help of object detection, the precise location of weeds from images can be achieved. The dataset is collected manually from a private farm in Gandhinagar, Gujarat, India. The combined approach of classification and object detection is applied in the proposed model. The Convolutional Neural Network (CNN) model is used to classify weed and non-weed images; further DL models are applied for object detection. We have compared DL models based on accuracy, memory usage, and Intersection over Union (IoU). ResNet-18, YOLOv3, CenterNet, and Faster RCNN are used in the proposed work. CenterNet outperforms all other models in terms of accuracy, i.e., 88%. Compared to other models, YOLOv3 is the least memory-intensive, utilizing 4.78 GB to evaluate the data.Keywords
Cite This Article
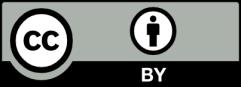