Open Access
ARTICLE
Convolutional LSTM Network for Heart Disease Diagnosis on Electrocardiograms
1 Department of Information Systems, Al-Farabi Kazakh National University, Almaty, Kazakhstan
2 Department of Mathematical and Computer Modeling, International Information Technology University, Almaty, Kazakhstan
3 Department of Software Engineering, Satbayev University, Almaty, Kazakhstan
* Corresponding Author: Batyrkhan Omarov. Email:
Computers, Materials & Continua 2023, 76(3), 3745-3761. https://doi.org/10.32604/cmc.2023.042627
Received 06 June 2023; Accepted 25 July 2023; Issue published 08 October 2023
Abstract
Heart disease is a leading cause of mortality worldwide. Electrocardiograms (ECG) play a crucial role in diagnosing heart disease. However, interpreting ECG signals necessitates specialized knowledge and training. The development of automated methods for ECG analysis has the potential to enhance the accuracy and efficiency of heart disease diagnosis. This research paper proposes a 3D Convolutional Long Short-Term Memory (Conv-LSTM) model for detecting heart disease using ECG signals. The proposed model combines the advantages of both convolutional neural networks (CNN) and long short-term memory (LSTM) networks. By considering both the spatial and temporal dependencies of ECG, the 3D Conv-LSTM model enables the detection of subtle changes in the signal over time. The model is trained on a dataset of ECG recordings from patients with various heart conditions, including arrhythmia, myocardial infarction, and heart failure. Experimental results show that the proposed 3D Conv-LSTM model outperforms traditional 2D CNN models in detecting heart disease, achieving an accuracy of 88% in the classification of five classes. Furthermore, the model outperforms the other state-of-the-art deep learning models for ECG-based heart disease detection. Moreover, the proposed Conv-LSTM network yields highly accurate outcomes in identifying abnormalities in specific ECG leads. The proposed 3D Conv-LSTM model holds promise as a valuable tool for automated heart disease detection and diagnosis. This study underscores the significance of incorporating spatial and temporal dependencies in ECG-based heart disease detection. It highlights the potential of deep-learning models in enhancing the accuracy and efficiency of diagnosis.Keywords
Cite This Article
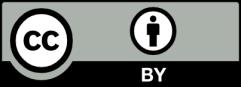
This work is licensed under a Creative Commons Attribution 4.0 International License , which permits unrestricted use, distribution, and reproduction in any medium, provided the original work is properly cited.