Open Access
ARTICLE
Improving Sentiment Analysis in Election-Based Conversations on Twitter with ElecBERT Language Model
1 School of Computer Science and Technology, Beijing Institute of Technology, Beijing, 100081, China
2 The Faculty of New Information and Communication Technologies, University Abdel-Hamid Mehri Constantine 2, Constantine, 25000, Algeria
3 Department of IT and Computer Science, Pak-Austria Fachhochschule: Institute of Applied Sciences and Technology, Haripur, 22620, Pakistan
* Corresponding Author: Huaping Zhang. Email:
(This article belongs to the Special Issue: Advance Machine Learning for Sentiment Analysis over Various Domains and Applications)
Computers, Materials & Continua 2023, 76(3), 3345-3361. https://doi.org/10.32604/cmc.2023.041520
Received 26 April 2023; Accepted 28 June 2023; Issue published 08 October 2023
Abstract
Sentiment analysis plays a vital role in understanding public opinions and sentiments toward various topics. In recent years, the rise of social media platforms (SMPs) has provided a rich source of data for analyzing public opinions, particularly in the context of election-related conversations. Nevertheless, sentiment analysis of election-related tweets presents unique challenges due to the complex language used, including figurative expressions, sarcasm, and the spread of misinformation. To address these challenges, this paper proposes Election-focused Bidirectional Encoder Representations from Transformers (ElecBERT), a new model for sentiment analysis in the context of election-related tweets. Election-related tweets pose unique challenges for sentiment analysis due to their complex language, sarcasm, and misinformation. ElecBERT is based on the Bidirectional Encoder Representations from Transformers (BERT) language model and is fine-tuned on two datasets: Election-Related Sentiment-Annotated Tweets (ElecSent)-Multi-Languages, containing 5.31 million labeled tweets in multiple languages, and ElecSent-English, containing 4.75 million labeled tweets in English. The model outperforms other machine learning models such as Support Vector Machines (SVM), Naïve Bayes (NB), and eXtreme Gradient Boosting (XGBoost), with an accuracy of 0.9905 and F1-score of 0.9816 on ElecSent-Multi-Languages, and an accuracy of 0.9930 and F1-score of 0.9899 on ElecSent-English. The performance of different models was compared using the 2020 United States (US) Presidential Election as a case study. The ElecBERT-English and ElecBERT-Multi-Languages models outperformed BERTweet, with the ElecBERT-English model achieving a Mean Absolute Error (MAE) of 6.13. This paper presents a valuable contribution to sentiment analysis in the context of election-related tweets, with potential applications in political analysis, social media management, and policymaking.Keywords
Cite This Article
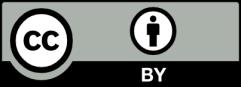
This work is licensed under a Creative Commons Attribution 4.0 International License , which permits unrestricted use, distribution, and reproduction in any medium, provided the original work is properly cited.